Develop and Test Local Azure Functions from your IDE
Offloading code from apps is a great way to adapt a microservices architecture. If you are still making the decision of whether to create functions or just code on your app, check out the decision matrix article and some gotchas that will help you know if you should create a function or not. Since we have checked the boxes and our code is a great candidate for Azure Functions then here’s our process:
Dev Environment Setup
Azure Functions Core Tools
First thing is to install the Azure Functions core tools on your machine. There are many ways to install the core tools and instructions can be found in the official Microsoft learn doc here: Develop Azure Functions locally using Core Tools | Microsoft Learn . We are using Ubuntu and Python so we did the following:
wget -q https://packages.microsoft.com/config/ubuntu/22.04/packages-microsoft-prod.deb
sudo dpkg -i packages-microsoft-prod.deb
Then:
sudo apt-get update
sudo apt-get install azure-functions-core-tools-4
After getting the core tools you can test by running
func --help
Result:
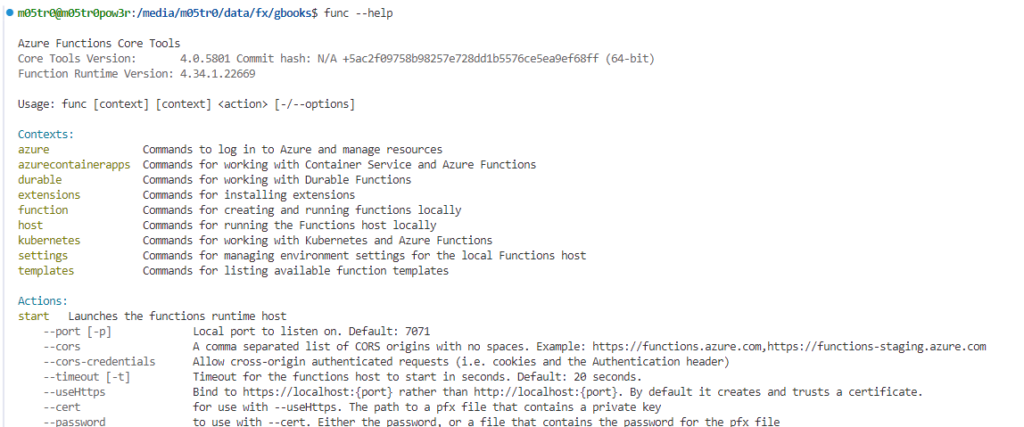
Visual Studio Code Extension
- Go to the Extensions view by clicking the Extensions icon in the Activity Bar.
- Search for “Azure Functions” and install the extension.
- Open the Command Palette (F1) and select
Azure Functions: Install or Update Azure Functions Core Tools
.
Azure Function Fundamentals
Here are some Azure Function Basics. You can write in many languages as described in the official Microsoft learn doc here: Supported Languages with Durable Functions Overview – Azure | Microsoft Learn . We are using Python so here’s our process
I. Create a Python Virtual Environment to manage dependencies:
A Python virtual environment is an isolated environment that allows you to manage dependencies for your project separately from other projects. Here are the key benefits:
- Dependency Isolation:
- Each project can have its own dependencies, regardless of what dependencies other projects have. This prevents conflicts between different versions of packages used in different projects.
- Reproducibility:
- By isolating dependencies, you ensure that your project runs consistently across different environments (development, testing, production). This makes it easier to reproduce bugs and issues.
- Simplified Dependency Management:
- You can easily manage and update dependencies for a specific project without affecting other projects. This is particularly useful when working on multiple projects simultaneously.
- Cleaner Development Environment:
- Your global Python environment remains clean and uncluttered, as all project-specific dependencies are contained within the virtual environment.
Create the virtual environment simply with: python -m venv name_of_venv
What is a Function Route?
A function route is essentially the path part of the URL that maps to your function. When an HTTP request matches this route, the function is executed. Routes are particularly useful for organizing and structuring your API endpoints.
II. Initialization
The line app = func.FunctionApp()
seen in the code snippet below is used in the context of Azure Functions for Python to create an instance of the FunctionApp
class. This instance, app
, serves as the main entry point for defining and managing your Azure Functions within the application. Here’s a breakdown of what it does:
- Initialization:
- It initializes a new
FunctionApp
object, which acts as a container for your function definitions.
- It initializes a new
- Function Registration:
- You use this
app
instance to register your individual functions. Each function is associated with a specific trigger (e.g., HTTP, Timer) and is defined using decorators.
- You use this
import azure.functions as func
app = func.FunctionApp()
@app.function_name(name="HttpTrigger1")
@app.route(route="hello")
def hello_function(req: func.HttpRequest) -> func.HttpResponse:
name = req.params.get('name')
if not name:
try:
req_body = req.get_json()
except ValueError:
pass
else:
name = req_body.get('name')
if name:
return func.HttpResponse(f"Hello, {name}!")
else:
return func.HttpResponse(
"Please pass a name on the query string or in the request body",
status_code=400
)
- The
@app.function_name
and@app.route
decorators are used to define the function’s name and route, respectively. This makes it easy to map HTTP requests to specific functions. - The
hello_function
is defined to handle HTTP requests. It extracts thename
parameter from the query string or request body and returns a greeting. - The function returns an
HttpResponse
object, which is sent back to the client.
What is a Function Route?
A function route is essentially the path part of the URL that maps to your function. When an HTTP request matches this route, the function is executed. Routes are particularly useful for organizing and structuring your API endpoints.
Running The Azure Function
Once you have your code ready to go you can test you function locally by using func start
but there are a few “gotchas” to be aware of:
1. Port Conflicts
- By default,
func start
runs on port 7071. If this port is already in use by another application, you’ll encounter a conflict. You can specify a different port using the--port
option:func start --port 8080
2. Environment Variables
- Ensure that all necessary environment variables are set correctly. Missing or incorrect environment variables can cause your function to fail. You can use a
local.settings.json
file to manage these variables during local development.
3. Dependencies
- Make sure all dependencies listed in your
requirements.txt
(for Python) orpackage.json
(for Node.js) are installed. Missing dependencies can lead to runtime errors.
4. Function Proxies
- If you’re using function proxies, ensure that the
proxies.json
file is correctly configured. Misconfigurations can lead to unexpected behavior or routing issues.
5. Binding Configuration
- Incorrect or incomplete binding configurations in your
function.json
file can cause your function to not trigger as expected. Double-check your bindings to ensure they are set up correctly.
6. Local Settings File
- The
local.settings.json
file should not be checked into source control as it may contain sensitive information. Ensure this file is listed in your.gitignore
file.
7. Cold Start Delays
- When running functions locally, you might experience delays due to cold starts, especially if your function has many dependencies or complex initialization logic.
8. Logging and Monitoring
- Ensure that logging is properly configured to help debug issues. Use the
func start
command’s output to monitor logs and diagnose problems.
9. Version Compatibility
- Ensure that the version of Azure Functions Core Tools you are using is compatible with your function runtime version. Incompatibilities can lead to unexpected errors.
10. Network Issues
- If your function relies on external services or APIs, ensure that your local environment has network access to these services. Network issues can cause your function to fail.
11. File Changes
- Be aware that changes to your function code or configuration files may require restarting the
func start
process to take effect.
12. Debugging
- When debugging, ensure that your IDE is correctly configured to attach to the running function process. Misconfigurations can prevent you from hitting breakpoints.
By keeping these gotchas in mind, you can avoid common pitfalls and ensure a smoother development experience with Azure Functions. If you encounter any specific issues or need further assistance, feel free to ask us!
Testing and Getting Results
If your function starts and you are looking at the logs you will see your endpoints listed as seen below but since you wrote them you know the paths as well and can start testing with your favorite API client, our favorite is Thunder Client.
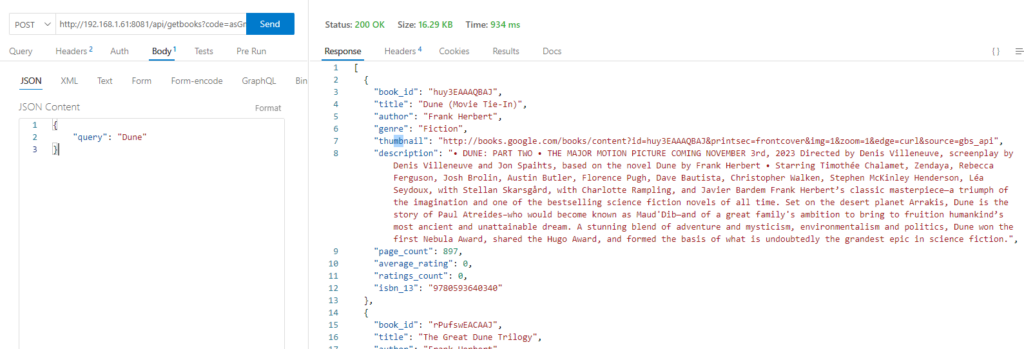
The Response
In Azure Functions, an HTTP response is what your function sends back to the client after processing an HTTP request. Here are the basics:
- Status Code:
- The status code indicates the result of the HTTP request. Common status codes include:
200 OK
: The request was successful.400 Bad Request
: The request was invalid.404 Not Found
: The requested resource was not found.500 Internal Server Error
: An error occurred on the server.
- The status code indicates the result of the HTTP request. Common status codes include:
- Headers:
- HTTP headers provide additional information about the response. Common headers include:
Content-Type
: Specifies the media type of the response (e.g.,application/json
,text/html
).Content-Length
: Indicates the size of the response body.Access-Control-Allow-Origin
: Controls which origins are allowed to access the resource.
- HTTP headers provide additional information about the response. Common headers include:
- Body:
- The body contains the actual data being sent back to the client. This can be in various formats such as JSON, HTML, XML, or plain text. We chose JSON so we can use the different fields and values.
Conclusion
In this article, we’ve explored the process of creating your first Python Azure Function using Visual Studio Code. We covered setting up your environment, including installing Azure Functions Core Tools and the VS Code extension, which simplifies project setup, development, and deployment. We delved into the importance of using a Python virtual environment and a requirements.txt
file for managing dependencies, ensuring consistency, and facilitating collaboration. Additionally, we discussed the basics of function routes and HTTP responses, highlighting how to define routes and customize responses to enhance your API’s structure and usability. By understanding these fundamentals, you can efficiently develop, test, and deploy serverless applications on Azure, leveraging the full potential of Azure Functions. Happy coding!
Free AI Inference with local Containers that leverage your NVIDIA GPU
First, let’s find out our GPU information from the OS perspective with the following command:
sudo lshw -C display
NVIDIA Drivers
Check your drivers are up to date so you can get the best features and security patches released. We are using ubuntu so will check by first
nvidia-smi
sudo modinfo nvidia | grep version
Then compare to see what’s in the apt repo to see if you have the latest with:
apt-cache search nvidia | grep nvidia-driver-5
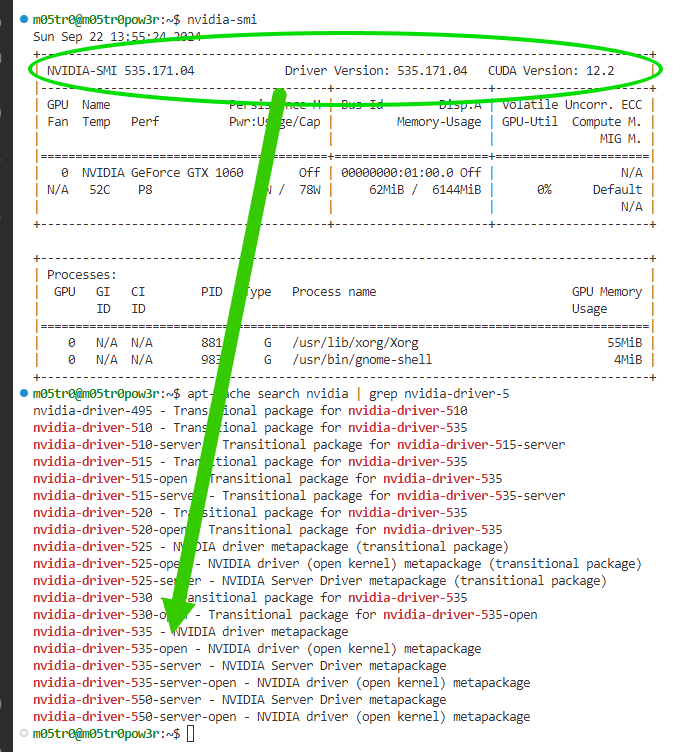
If this is your first time installing drivers please see:
Configure the NVIDIA Toolkit Runtime for Docker
nvidia-ctk is a command-line tool you get when you configure the NVIDIA Container Toolkit. It’s used to configure and manage the container runtime (Docker or containerd) to enable GPU support within containers. To configure you can simply run the following
sudo nvidia-ctk runtime configure --runtime=docker
sudo systemctl restart docker
Here are some of its primary functions:
- Configuring runtime: Modifies the configuration files of Docker or containerd to include the NVIDIA Container Runtime.
- Generating CDI specifications: Creates configuration files for the Container Device Interface (CDI), which allows containers to access GPU devices.
- Listing CDI devices: Lists the available GPU devices that can be used by containers.
In essence, nvidia-ctk acts as a bridge between the container runtime and the NVIDIA GPU, ensuring that containers can effectively leverage GPU acceleration.
Tip: In cases where you want to split one GPU you could create multiple CDI devices which are virtual slices of the GPU. Say you have a GPU with 6GB of RAM, you could create 2 devices with the nvidia-ctk command like so:
nvidia-ctk create-cdi --device-path /dev/nvidia0 --device-id 0 --memory 2G --name cdi1
nvidia-ctk create-cdi --device-path /dev/nvidia0 --device-id 0 --memory 4G --name cdi2
Now you can assign each to containers to limit their utilization of the GPU ram like this:
docker run --gpus device=cdi1,cdi2
Run Containers with GPUs
After configuring the Driver and NVIDIA Container Toolkit you are ready to run GPU-powered containers. One of our favorites is the Ollama containers that allow you to run AI Inference endpoints.
docker run -it --rm --gpus=all -v /home/ollama:/root/.ollama:z -p 11434:11434 --name ollama ollama/ollama
Notice we are using all gpus in this instance.
Sources:
Django Microservices Approach with Azure Functions on Azure Container Apps
We are creating a multi-part video to explain Azure Functions running on Azure Container Apps so that we can offload some of the code out of our Django App and build our infrastructure with a microservice approach. Here’s part one and below the video a quick high-level explanation for this architecture.
Azure Functions are serverless computing units within Azure that allow you to run event-driven code without having to manage servers. They’re a great choice for building microservices due to their scalability, flexibility, and cost-effectiveness.
Azure Container Apps provide a fully managed platform for deploying and managing containerized applications. By deploying Azure Functions as containerized applications on Container Apps, you gain several advantages:
-
Microservices Architecture:
- Decoupling: Each function becomes an independent microservice, isolated from other parts of your application. This makes it easier to develop, test, and deploy them independently.
- Scalability: You can scale each function individually based on its workload, ensuring optimal resource utilization.
- Resilience: If one microservice fails, the others can continue to operate, improving the overall reliability of your application.
-
Containerization:
- Portability: Containerized functions can be easily moved between environments (development, testing, production) without changes.
- Isolation: Each container runs in its own isolated environment, reducing the risk of conflicts between different functions.
- Efficiency: Containers are optimized for resource utilization, making them ideal for running functions on shared infrastructure.
-
Azure Container Apps Benefits:
- Managed Service: Azure Container Apps handles the underlying infrastructure, allowing you to focus on your application’s logic.
- Scalability: Container Apps automatically scale your functions based on demand, ensuring optimal performance.
- Integration: It seamlessly integrates with other Azure services, such as Azure Functions, Azure App Service, and Azure Kubernetes Service.
In summary, Azure Functions deployed on Azure Container Apps provide a powerful and flexible solution for building microservices. By leveraging the benefits of serverless computing, containerization, and a managed platform, you can create scalable, resilient, and efficient applications.
Stay tuned for part 2
Deploying Azure Functions with Azure DevOps: 3 Must-Dos! Code Security Included
Azure Functions is a serverless compute service that allows you to run your code in response to various events, without the need to manage any infrastructure. Azure DevOps, on the other hand, is a set of tools and services that help you build, test, and deploy your applications more efficiently. Combining these two powerful tools can streamline your Azure Functions deployment process and ensure a smooth, automated workflow.
In this blog post, we’ll explore three essential steps to consider when deploying Azure Functions using Azure DevOps.
1. Ensure Consistent Python Versions
When working with Azure Functions, it’s crucial to ensure that the Python version used in your build pipeline matches the Python version configured in your Azure Function. Mismatched versions can lead to unexpected runtime errors and deployment failures.
To ensure consistency, follow these steps:
- Determine the Python version required by your Azure Function. You can find this information in the
requirements.txt
file or thehost.json
file in your Azure Functions project. - In your Azure DevOps pipeline, use the
UsePythonVersion
task to set the Python version to match the one required by your Azure Function.
- task: UsePythonVersion@0
inputs:
versionSpec: '3.9'
addToPath: true
- Verify the Python version in your pipeline by running
python --version
and ensuring it matches the version specified in the previous step.
2. Manage Environment Variables Securely
Azure Functions often require access to various environment variables, such as database connection strings, API keys, or other sensitive information. When deploying your Azure Functions using Azure DevOps, it’s essential to handle these environment variables securely.
Here’s how you can approach this:
- Store your environment variables as Azure DevOps Service Connections or Azure Key Vault Secrets.
- In your Azure DevOps pipeline, use the appropriate task to retrieve and set the environment variables. For example, you can use the
AzureKeyVault
task to fetch secrets from Azure Key Vault.
- task: AzureKeyVault@1
inputs:
azureSubscription: 'Your_Azure_Subscription_Connection'
KeyVaultName: 'your-keyvault-name'
SecretsFilter: '*'
RunAsPreJob: false
- Ensure that your pipeline has the necessary permissions to access the Azure Key Vault or Service Connections.
3. Implement Continuous Integration and Continuous Deployment (CI/CD)
To streamline the deployment process, it’s recommended to set up a CI/CD pipeline in Azure DevOps. This will automatically build, test, and deploy your Azure Functions whenever changes are made to your codebase.
Here’s how you can set up a CI/CD pipeline:
- Create an Azure DevOps Pipeline and configure it to trigger on specific events, such as a push to your repository or a pull request.
- In the pipeline, include steps to build, test, and package your Azure Functions project.
- Add a deployment task to the pipeline to deploy your packaged Azure Functions to the target Azure environment.
# CI/CD pipeline
trigger:
- main
pool:vmImage: ‘ubuntu-latest’
steps:– task: UsePythonVersion@0
inputs:
versionSpec: ‘3.9’
addToPath: true
– script: |pip install -r requirements.txt
displayName: ‘Install dependencies’
– task: AzureWebApp@1inputs:
azureSubscription: ‘Your_Azure_Subscription_Connection’
appName: ‘your-function-app-name’
appType: ‘functionApp’
deployToSlotOrASE: true
resourceGroupName: ‘your-resource-group-name’
slotName: ‘production’
By following these three essential steps, you can ensure a smooth and reliable deployment of your Azure Functions using Azure DevOps, maintaining consistency, security, and automation throughout the process.
Bonus: Embrace DevSecOps with Code Security Checks
As part of your Azure DevOps pipeline, it’s crucial to incorporate security checks to ensure the integrity and safety of your code. This is where the principles of DevSecOps come into play, where security is integrated throughout the software development lifecycle.
Here’s how you can implement code security checks in your Azure DevOps pipeline:
- Use Bandit for Python Code Security: Bandit is a popular open-source tool that analyzes Python code for common security issues. You can integrate Bandit into your Azure DevOps pipeline to automatically scan your Azure Functions code for potential vulnerabilities.
- script: |
pip install bandit
bandit -r your-functions-directory -f custom -o bandit_report.json
displayName: 'Run Bandit Security Scan'
- task: PublishBuildArtifacts@1
inputs:
PathtoPublish: 'bandit_report.json'
ArtifactName: 'bandit-report'
publishLocation: 'Container'
- Leverage the Safety Tool for Dependency Scanning: Safety is another security tool that checks your Python dependencies for known vulnerabilities. Integrate this tool into your Azure DevOps pipeline to ensure that your Azure Functions are using secure dependencies.
- script: |
pip install safety
safety check --full-report
displayName: 'Run Safety Dependency Scan'
- Review Security Scan Results: After running the Bandit and Safety scans, review the generated reports and address any identified security issues before deploying your Azure Functions. You can publish the reports as build artifacts in Azure DevOps for easy access and further investigation.
By incorporating these DevSecOps practices into your Azure DevOps pipeline, you can ensure that your Azure Functions are not only deployed efficiently but also secure and compliant with industry best practices.
The AI-Driven Evolution of Databases
The hype of Artificial Intelligence (AI) and Retrieval-Augmented Generation (RAG) is revolutionizing databases and how they are architected. Traditional database management systems (DBMS) are being redefined to harness the capabilities of AI, transforming how data is stored, retrieved, and utilized. In this article I am sharing some of the shifts happening right now to catch up and create better DBs that can play nice with AI.
1. Vectorization and Embedding Integration
Traditional databases store data in structured formats, typically as rows and columns in tables. However, with the rise of AI, there is a need to store and query high-dimensional data such as vectors (embeddings), which represent complex data types like images, audio, and natural language.
- Embedding Vectors: When new data is inserted into the database, it can be vectorized using machine learning models, converting the data into embedding vectors. This allows for efficient similarity searches and comparisons. For example, inserting a new product description could automatically generate an embedding that captures its semantic meaning.
- Vector Databases: Specialized vector databases like Pinecone, Weaviate, FAISS (Facebook AI Similarity Search) and Azure AI Search are designed to handle and index vectorized data, enabling fast and accurate similarity searches and nearest neighbor queries.
A great example is PostgreSQL which can be extended to handle high-dimensional vector data efficiently using the pgvector
extension. This capability is particularly useful for applications involving machine learning, natural language processing, and other AI-driven tasks that rely on vector representations of data.
What is pgvector?
pgvector
is an extension for PostgreSQL that enables the storage, indexing, and querying of vector data. Vectors are often used to represent data in a high-dimensional space, such as word embeddings in NLP, feature vectors in machine learning, and image embeddings in computer vision.
2. Enhanced Indexing Techniques
One of the main changes to support AI is that now your index is required to support ANN (approximate nearest neighbor) queries against vector data. A typical query would be “find me the top N vectors that are most similar to this one”. Each vector may have 100s or 1000s of dimensions, and similarity is based on overall distance across all these dimensions. Your regular btree or hash table index is completely useless for this kind of query, so new types of indexes are provided as part of pgvector on PostgreSQL, or you could use Pinecone, Milvus and many solutions being developed as AI keeps demanding data, these solutions are more specialized for these workloads.
Databases are adopting hybrid indexing techniques that combine traditional indexing methods (B-trees, hash indexes) with AI-driven indexes such as neural hashes and inverted indexes for text and multimedia data.
- AI-Driven Indexing: Machine learning algorithms can optimize index structures by predicting access patterns and preemptively loading relevant data into memory, reducing query response times.
What is an Approximate Nearest Neighbor (ANN) Search? It’s an algorithm that finds a data point in a data set that’s very close to the given query point, but not necessarily the absolute closest one. An NN algorithm searches exhaustively through all the data to find the perfect match, whereas an ANN algorithm will settle for a match that’s close enough.
3. Automated Data Management and Maintenance
AI-driven databases can automatically adjust configurations and optimize performance based on workload analysis. This includes automatic indexing, query optimization, and resource allocation.
- Adaptive Query Optimization: AI models predict the best execution plans for queries by learning from historical data, continuously improving query performance over time.
Predictive Maintenance: Machine learning models can predict hardware failures and performance degradation, allowing for proactive maintenance and minimizing downtime.
Some examples:
- Azure SQL Database offers built-in AI features such as automatic tuning, which includes automatic indexing and query performance optimization. Azure DBs also provide insights with machine learning to analyze database performance and recommend optimizations.
- Google BigQuery incorporates machine learning to optimize query execution and manage resources efficiently and allows users to create and execute machine learning models directly within the database.
- Amazon Aurora utilizes machine learning to optimize queries, predict database performance issues, and automate database management tasks such as indexing and resource allocation. They also integrate machine learning capabilities directly into the database, allowing for real-time predictions and automated adjustments.
Wrap-Up
The landscape of database technology is rapidly evolving, driven by the need to handle more complex data types, improve performance, and integrate seamlessly with machine learning workflows. Innovations like vectorization during inserts, enhanced indexing techniques, and automated data management are at the forefront of this transformation. As these technologies continue to mature, databases will become even more powerful, enabling new levels of efficiency, intelligence, and security in data management.
Unleash Your Creativity with NVIDIA Jetson Nano: The Ultimate AI Home Lab SBC
If you are looking to practice with machine learning, AI or deep learning without the cloud costs, then look no further than the NVIDIA Jetson Nano, your ticket to unlocking endless possibilities in AI development and innovation. In this quick blog article, we’ll explore some of the remarkable features and benefits of the NVIDIA Jetson Nano and how it can revolutionize your AI projects.
Powerful Performance in a Compact Package
Don’t let its small size fool you – the NVIDIA Jetson Nano packs a punch when it comes to performance. Powered by the NVIDIA CUDA-X AI computing platform, this tiny yet mighty device delivers exceptional processing power for AI workloads. With its quad-core ARM Cortex-A57 CPU and 128-core NVIDIA Maxwell GPU, the Jetson Nano is capable of handling complex AI tasks with ease, whether it’s image recognition, natural language processing, or autonomous navigation.
Here are the full specs: https://developer.nvidia.com/embedded/jetson-nano
One drawback is that the supported image is on Ubuntu 18.04 and there is no support to upgrade.
Easy to Use and Versatile
One of the standout features of the NVIDIA Jetson Nano is its user-friendly design. Whether you’re a seasoned AI developer or a beginner just getting started, the Jetson Nano is incredibly easy to set up and use. Thanks to its comprehensive documentation and extensive developer community, you’ll be up and running in no time, ready to unleash your creativity and bring your AI projects to life.
Here are some links to get started:
https://developer.nvidia.com/embedded/learn/get-started-jetson-nano-devkit
https://developer.nvidia.com/embedded/learn/jetson-ai-certification-programs
Endless Possibilities for Innovation
The NVIDIA Jetson Nano opens up a world of possibilities for AI innovation. Whether you’re building a smart robot, creating intelligent IoT devices, or developing groundbreaking machine learning applications, the Jetson Nano provides the tools and resources you need to succeed. With support for popular AI frameworks like TensorFlow, PyTorch, and Caffe, as well as compatibility with a wide range of sensors and peripherals, the Jetson Nano gives you the freedom to explore and experiment like never before.
Here’s a link to see projects:
https://developer.nvidia.com/embedded/community/jetson-projects?refinementList%5Bworks_with%5D%5B0%5D=Jetson%20Nano%202GB&refinementList%5Bworks_with%5D%5B1%5D=Jetson%20Nano&page=1
Understanding GPU Evolution and Deployment in Datacenter or Cloud
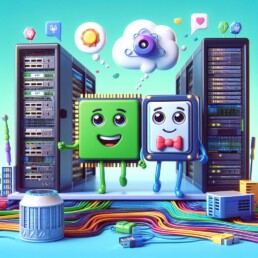
In the current hype of Artificial Intelligence we find ourselves working a lot more with GPUs which is very fun! GPU workloads can be run on physical servers, VMs, or containers. Each approach has its own advantages and disadvantages.
Physical servers offer the highest performance and flexibility, but they are also the most expensive and complex to manage. VMs provide a good balance between performance, flexibility, resource utilization, and cost. Containers offer the best resource utilization and cost savings, but they may sacrifice some performance and isolation.
For Cloud we often recommend Containers since there are services where you can spin up, run your jobs and spin down. A Physical VM running 24-7 on cloud services can be expensive. The performance trade-off is often solved by distributing load or development.
The best deployment option for your GPU workloads will depend on your specific needs and requirements. If you need the highest possible performance and flexibility, physical servers are the way to go. If you are looking to improve resource utilization and cost savings, VMs or containers may be a better option.
Deployment | Description | Benefits | Disadvantages |
---|---|---|---|
Physical servers | Each GPU is directly dedicated to a single workload. | Highest performance and flexibility. | Highest cost and complexity. |
VMs | GPUs can be shared among multiple workloads. | Improved resource utilization and cost savings. | Reduced performance and flexibility. |
Containers | GPUs can be shared among multiple workloads running in the same container host. | Improved resource utilization and cost savings. | Reduced performance and isolation. |
Additional considerations:
- GPU virtualization: GPU virtualization technologies such as NVIDIA vGPU and AMD SR-IOV allow you to share a single physical GPU among multiple VMs or containers. This can improve resource utilization and reduce costs, but it may also reduce performance.
- Workload type: Some GPU workloads are more sensitive to performance than others. For example, machine learning and artificial intelligence workloads often require the highest possible performance. Other workloads, such as video encoding and decoding, may be more tolerant of some performance degradation.
- Licensing: Some GPU software applications are only licensed for use on physical servers. If you need to use this type of software, you will need to deploy your GPU workloads on physical servers.
Overall, the best way to choose the right deployment option for your GPU workloads is to carefully consider your specific needs and requirements.
Here is a table summarizing the key differences and benefits of each deployment options:
Characteristic | Physical server | VM | Container |
---|---|---|---|
Performance | Highest | High | Medium |
Flexibility | Highest | High | Medium |
Resource utilization | Medium | High | Highest |
Cost | Highest | Medium | Lowest |
Complexity | Highest | Medium | Lowest |
Isolation | Highest | High | Medium |
Building Windows Servers with Hashicorp Packer + Terraform on Oracle Cloud Infrastructure OCI
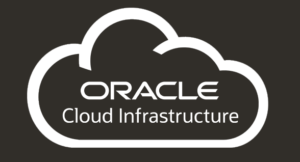
In today’s dynamic IT landscape, platform engineers juggle a diverse array of cloud technologies to cater to specific client needs. Among these, Oracle Cloud Infrastructure (OCI) is rapidly gaining traction due to its competitive pricing for certain services. However, navigating the intricacies of each cloud can present a significant learning curve. This is where cloud-agnostic tools like Terraform and Packer shine. By abstracting away the underlying APIs and automating repetitive tasks, they empower us to leverage OCI’s potential without getting bogged down in vendor-specific complexities.
In this article I show you how to get started with Oracle Cloud by using Packer and Terraform for Windows servers, and this can be used for other Infrastructure as code tasks.
Oracle Cloud Infrastructure Configs
OCI Keys for API Use
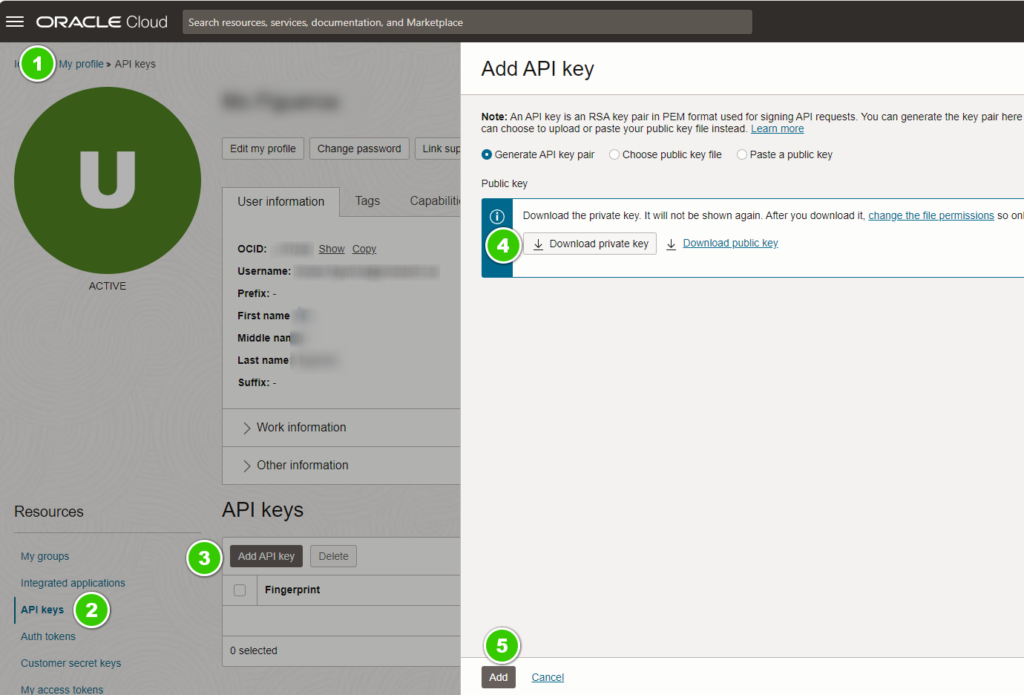
Prerequisite: Before you generate a key pair, create the .oci
directory in your home directory to store the credentials. See SDK and CLI Configuration File for more details.
- View the user’s details:
- If you’re adding an API key for yourself:
Open the Profile menu and click My profile.
- If you’re an administrator adding an API key for another user: Open the navigation menu and click Identity & Security. Under Identity, click Users. Locate the user in the list, and then click the user’s name to view the details.
- If you’re adding an API key for yourself:
- In the Resources section at the bottom left, click API Keys
- Click Add API Key at the top left of the API Keys list. The Add API Key dialog displays.
-
Click Download Private Key and save the key to your
.oci
directory. In most cases, you do not need to download the public key.Note: If your browser downloads the private key to a different directory, be sure to move it to your
.oci
directory. - Click Add.
The key is added and the Configuration File Preview is displayed. The file snippet includes required parameters and values you’ll need to create your configuration file. Copy and paste the configuration file snippet from the text box into your
~/.oci/config file
. (If you have not yet created this file, see SDK and CLI Configuration File for details on how to create one.)After you paste the file contents, you’ll need to update the
key_file
parameter to the location where you saved your private key file.If your config file already has a DEFAULT profile, you’ll need to do one of the following:
- Replace the existing profile and its contents.
- Rename the existing profile.
- Rename this profile to a different name after pasting it into the config file.
- Update the permissions on your downloaded private key file so that only you can view it:
- Go to the
.oci
directory where you placed the private key file. - Use the command
chmod go-rwx ~/.oci/<oci_api_keyfile>.pem
to set the permissions on the fil
- Go to the
Network
Make sure to allow WinRM and RDP so that packer can configure the VM and make it into an image and so that you can RDP to the server after it’s created.
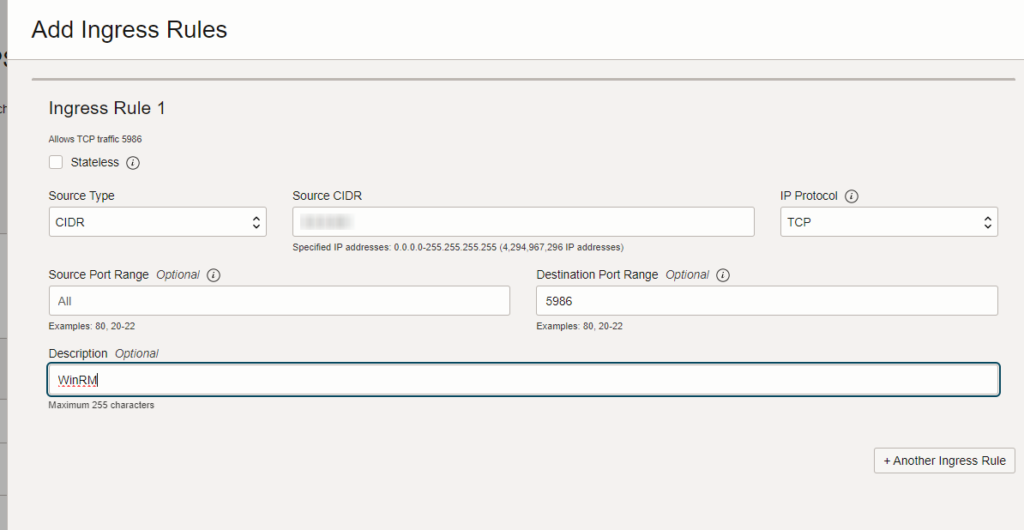
Packer Configuration & Requirements
Install the packer OCI plugin on the host running packer
$ packer plugins install github.com/hashicorp/oracle
Packer Config
- Configure your source
- Availability domain:
oci iam availability-domain list
- Availability domain:
- Get your base image (Drivers Included)
- With the OCI cli:
oci compute image list --compartment-id "ocid#.tenancy.XXXX" --operating-system "Windows" | grep -e 2019 -e ocid1
- With the OCI cli:
- Point to config file that has the OCI Profile we downloaded in the previous steps.
- WinRM Config
- User Data (Bootstrap)
- You must set the password to not be changed at next logon so that packer can connect:
- Code:
#ps1_sysnative
cmd /C 'wmic UserAccount where Name="opc" set PasswordExpires=False'
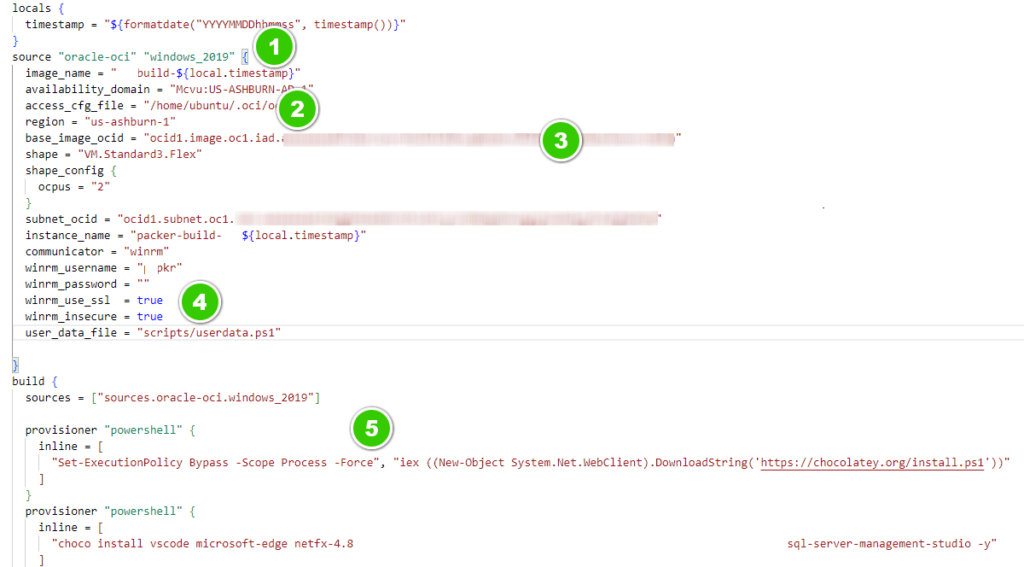
Automating Special Considerations from OCI
Images can be used to launch other instances. The instances launched from these images will include the customizations, configurations, and software installed when the image was created. For windows a we need to sysprep but OCI has specifics on doing so.
Creating a generalized image from an instance will render the instance non-functional, so you should first create a custom image from the instance, and then create a new instance from the custom image. Source below
We automated their instruction by:
- Extract the contents of oracle-cloud_windows-server_generalize_2022-08-24.SED.EXE to your packer scripts directory
- Copy all files to C:\Windows\Panther
- Use the windows-shell provisioner in packer to run Generalize.cmd
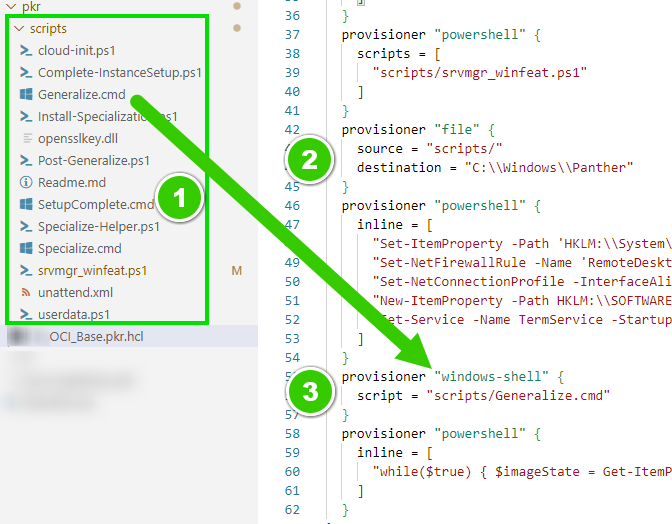
Terraform Config with Oracle Cloud
- Configure the vars
Oracle OCI Terraform Variables - Pass the private key at runtime:
terraform apply --var-file=oci.tfvars -var=private_key_path=~/.oci/user_2024-10-30T10_10_10.478Z.pem
Sources:
Sys-prepping in OCI is specific to their options here’s a link:
https://docs.oracle.com/en-us/iaas/Content/Compute/References/windowsimages.htm#Windows_Generalized_Image_Support_Files
Other Sources:
https://docs.oracle.com/en-us/iaas/Content/API/Concepts/apisigningkey.htm#apisigningkey_topic_How_to_Generate_an_API_Signing_Key_Console
https://github.com/hashicorp/packer/issues/7033
https://github.com/hashicorp/packer-plugin-oracle/tree/main/docs
Avoid Full Downtime on Auto-Scaled Environments by Only Targeting New Instances with Ansible and Github Actions!
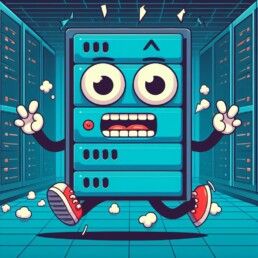
Servers are scared of downtime!
The following Ansible playbook provides a simple but powerful way to compare instance uptime to a threshold “scale_time” variable that you can set in your pipeline variables in Github. By checking the uptime, you can selectively run tasks only on machines newer than that time period you set to avoid downtime on the rest.
Of course the purpose of Ansible is to be idempotent but sometimes during testing we might need to isolate servers to not affect all, specially when using dynamic inventories.
Solution: The Playbook
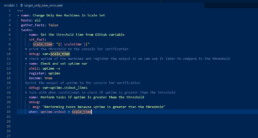
How it works:
- Create a variable in Github Pipeline Variables.
- Set the variable at runtime:
ansible-playbook -i target_only_new_vmss.yml pm2status.yml -e "scaletime=${{ vars.SCALETIME }}"
- The
set_fact
task defines thescale_time
variable based on when the last scaling event occurred. This will be a timestamp. - The
uptime
command gets the current uptime of the instance. This is registered as a variable. - Using a conditional
when
statement, we only run certain tasks if the uptime is less than thescale_time
threshold. - This allows you to selectively target new instances created after the last scale-up event.
Benefits:
- Avoid unnecessary work on stable instances that don’t need updates.
- Focus load and jobs on new machines only
- Safer rollouts in large auto-scaled environments by targeting smaller batches.
- Easy way to check uptime against a set point in time.
Leveraging GitHub Actions for Efficient Infrastructure Automation with Separate Workflows.
Building infrastructure requires a well-defined pipeline. This article demonstrates how to leverage GitHub Actions to build an Amazon Machine Image (AMI) with Packer and then automatically trigger a separate Terraform workflow via Github’s Workflow API and pass the AMI ID as well.
Benefits:
- Streamlined workflow: Packer builds the AMI, and the AMI ID is seamlessly passed to the Terraform workflow for deployment.
- Reduced manual intervention: The entire process is automated, eliminating the need to manually trigger the Terraform workflow or update the AMI ID.
- Improved efficiency: Faster deployment cycles and reduced risk of errors due to manual configuration.
Why separate workflows?
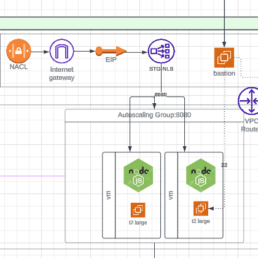
First, think about a simple AWS architecture consisting on a Load Balancer in front of an Autoscaling group, you still need to build a VM image, make sure the load balancer has 2 networks for HA and add security groups for layer 4 access controls. The VM will be built by packer and terraform will deploy the rest of the components so your workflow consists of 2 jobs Packer builds, Terraform deploys but I am here to challenge this approach. You might think this goes against Build / Deploy workflows since most workflows or pipelines have the 2 job pattern of packer build then Terraform deploys but often times we see that we need to separate them because the work we do in Terraform is separate and shouldn’t depend on building an AMI every time.
Think of updating the number of machines on the scale set. Doing it manually will cause drift and the typical workflow will need to run packer before getting to Terraform which is not too bad but we are wasting some cycles.
Separating the workflows makes more sense because you can run terraform to update your infrastructure components from any API Client. Having Terraform in a separate workflow gets rid of the dependency of running packer every time. Ultimately, the choice between the two methods depends on your specific requirements and preferences.
Build and Trigger the Next Workflow
In the packer workflow we add a second job to trigger terraform. We have to pass our Personal Access Token (PAT) and the AMI_ID so that terraform can update the VM Autoscaling Group.
trigger_another_repo:
needs: packer
runs-on: ubuntu-latest
steps:
- name: Trigger second workflow
env:
AMITF: ${{ needs.packer.outputs.AMI_ID_TF }}
run: |
curl -X POST \
-H "Authorization: token ${{ secrets.PAT }}" \
-H "Accept: application/vnd.github.everest-preview+json" \
"https://api.github.com/repos/repo_name/workflow_name/dispatches" \
-d '{"event_type": "trigger_tf_build", "client_payload": {"variable_name": "${{ needs.packer.outputs.AMI_ID_TF }}"}}'
As you can see we are simply using CURL to send the data payload to the Terraform workflow.
The Triggered Workflow Requirements
For the Terraform workflow to start from the packer trigger we need a few simple things.
- Workflow trigger
on:
repository_dispatch:
types: [trigger_prod_tf_build]
- Confirm variable (Optional)
- name: Print Event Payload
run: echo "${{ github.event.client_payload.variable_name }}"
While combining Packer and Terraform into a single workflow can simplify things in certain scenarios, separating them provides more granular control, reusability, and scalability. The best approach depends on the specific needs and complexity of your infrastructure.