OpenWebUI & Ollama: Experience AI on Your Terms with Local Hosting
In an era where AI solutions are behind subscription models behind cloud-based solutions, OpenWebUI and Ollama provide a powerful alternative that prioritize privacy, security, and cost efficiency. These open-source tools are revolutionizing how organizations and individuals can harness AI capabilities while maintaining complete control of models and data used.
Why use local LLMs? #1 Uncensored Models
One significant advantage of local deployment through Ollama is the ability to use a model of your choosing which includes unrestricted LLMs. While cloud-based AI services often implement various limitations and filters on their models to maintain content control and reduce liability, locally hosted models can be used without these restrictions. This provides several benefits:
- Complete control over model behavior and outputs
- Ability to fine-tune models for specific use cases without limitations
- Access to open-source models with different training approaches
- Freedom to experiment with model parameters and configurations
- No artificial constraints on content generation or topic exploration
This flexibility is particularly valuable for research, creative applications, and specialized industry use cases where standard content filters might interfere with legitimate work.
Here’s an amazing article from Eric Hartford on: Uncensored Models
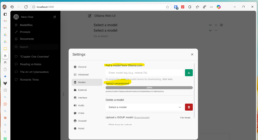
Why use local LLMs? #2 Privacy
When running AI models locally through Ollama and OpenWebUI, all data processing occurs on your own infrastructure. This means:
- Sensitive data never leaves your network perimeter
- No third-party access to your queries or responses
- Complete control over data retention and deletion policies
- Compliance with data sovereignty requirements
- Protection from cloud provider data breaches
Implementation
Requirements:
- Docker
- NVIDIA Container Toolkit (Optional but Recommended)
- GPU + NVIDIA Cuda Installation (Optional but Recommended)
Step 1: Install Ollama
docker run -d -v ollama:/root/.ollama -p 11434:11434 --name ollama ollama/ollama:latest
Step 2: Launch Open WebUI with the new features
docker run -d -p 3000:8080 --add-host=host.docker.internal:host-gateway -v open-webui:/app/backend/data --name open-webui --restart always ghcr.io/open-webui/open-webui:main
Need help setting up Docker and Nvidia Container toolkit?
OpenWeb UI and Ollama
OpenWebUI provides a sophisticated interface for interacting with locally hosted models while maintaining all the security benefits of local deployment. Key features include:
- Intuitive chat interface similar to popular cloud-based AI services
- Support for multiple concurrent model instances
- Built-in prompt templates and history management
- Customizable UI themes and layouts
- API integration capabilities for internal applications
Ollama simplifies the process of running AI models locally while providing robust security features:
- Easy model installation and version management
- Efficient resource utilization through optimized inference
- Support for custom model configurations
- Built-in model verification and integrity checking
- Container-friendly architecture for isolated deployments
Free AI Inference with local Containers that leverage your NVIDIA GPU
First, let’s find out our GPU information from the OS perspective with the following command:
sudo lshw -C display
NVIDIA Drivers
Check your drivers are up to date so you can get the best features and security patches released. We are using ubuntu so will check by first
nvidia-smi
sudo modinfo nvidia | grep version
Then compare to see what’s in the apt repo to see if you have the latest with:
apt-cache search nvidia | grep nvidia-driver-5
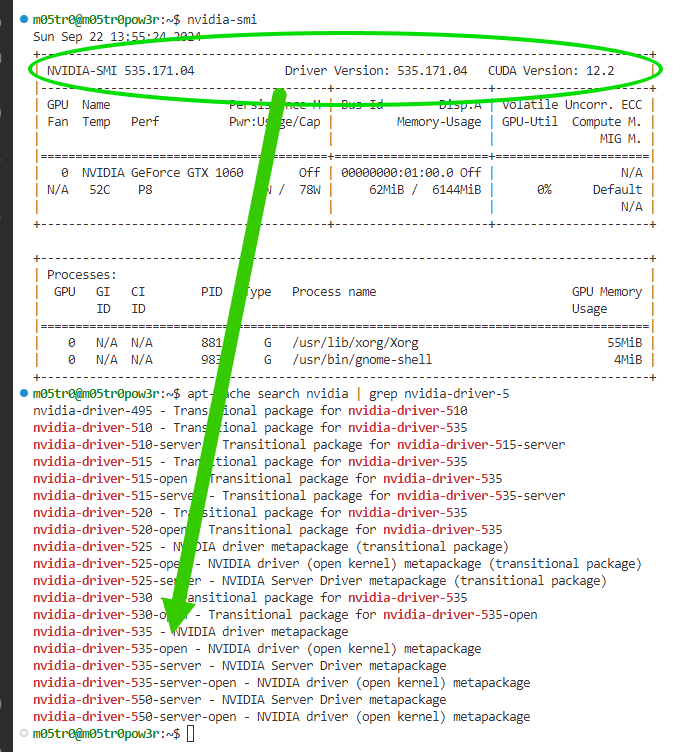
If this is your first time installing drivers please see:
Configure the NVIDIA Toolkit Runtime for Docker
nvidia-ctk is a command-line tool you get when you configure the NVIDIA Container Toolkit. It’s used to configure and manage the container runtime (Docker or containerd) to enable GPU support within containers. To configure you can simply run the following
sudo nvidia-ctk runtime configure --runtime=docker
sudo systemctl restart docker
Here are some of its primary functions:
- Configuring runtime: Modifies the configuration files of Docker or containerd to include the NVIDIA Container Runtime.
- Generating CDI specifications: Creates configuration files for the Container Device Interface (CDI), which allows containers to access GPU devices.
- Listing CDI devices: Lists the available GPU devices that can be used by containers.
In essence, nvidia-ctk acts as a bridge between the container runtime and the NVIDIA GPU, ensuring that containers can effectively leverage GPU acceleration.
Tip: In cases where you want to split one GPU you could create multiple CDI devices which are virtual slices of the GPU. Say you have a GPU with 6GB of RAM, you could create 2 devices with the nvidia-ctk command like so:
nvidia-ctk create-cdi --device-path /dev/nvidia0 --device-id 0 --memory 2G --name cdi1
nvidia-ctk create-cdi --device-path /dev/nvidia0 --device-id 0 --memory 4G --name cdi2
Now you can assign each to containers to limit their utilization of the GPU ram like this:
docker run --gpus device=cdi1,cdi2
Run Containers with GPUs
After configuring the Driver and NVIDIA Container Toolkit you are ready to run GPU-powered containers. One of our favorites is the Ollama containers that allow you to run AI Inference endpoints.
docker run -it --rm --gpus=all -v /home/ollama:/root/.ollama:z -p 11434:11434 --name ollama ollama/ollama
Notice we are using all gpus in this instance.
Sources:
The AI-Driven Evolution of Databases
The hype of Artificial Intelligence (AI) and Retrieval-Augmented Generation (RAG) is revolutionizing databases and how they are architected. Traditional database management systems (DBMS) are being redefined to harness the capabilities of AI, transforming how data is stored, retrieved, and utilized. In this article I am sharing some of the shifts happening right now to catch up and create better DBs that can play nice with AI.
1. Vectorization and Embedding Integration
Traditional databases store data in structured formats, typically as rows and columns in tables. However, with the rise of AI, there is a need to store and query high-dimensional data such as vectors (embeddings), which represent complex data types like images, audio, and natural language.
- Embedding Vectors: When new data is inserted into the database, it can be vectorized using machine learning models, converting the data into embedding vectors. This allows for efficient similarity searches and comparisons. For example, inserting a new product description could automatically generate an embedding that captures its semantic meaning.
- Vector Databases: Specialized vector databases like Pinecone, Weaviate, FAISS (Facebook AI Similarity Search) and Azure AI Search are designed to handle and index vectorized data, enabling fast and accurate similarity searches and nearest neighbor queries.
A great example is PostgreSQL which can be extended to handle high-dimensional vector data efficiently using the pgvector
extension. This capability is particularly useful for applications involving machine learning, natural language processing, and other AI-driven tasks that rely on vector representations of data.
What is pgvector?
pgvector
is an extension for PostgreSQL that enables the storage, indexing, and querying of vector data. Vectors are often used to represent data in a high-dimensional space, such as word embeddings in NLP, feature vectors in machine learning, and image embeddings in computer vision.
2. Enhanced Indexing Techniques
One of the main changes to support AI is that now your index is required to support ANN (approximate nearest neighbor) queries against vector data. A typical query would be “find me the top N vectors that are most similar to this one”. Each vector may have 100s or 1000s of dimensions, and similarity is based on overall distance across all these dimensions. Your regular btree or hash table index is completely useless for this kind of query, so new types of indexes are provided as part of pgvector on PostgreSQL, or you could use Pinecone, Milvus and many solutions being developed as AI keeps demanding data, these solutions are more specialized for these workloads.
Databases are adopting hybrid indexing techniques that combine traditional indexing methods (B-trees, hash indexes) with AI-driven indexes such as neural hashes and inverted indexes for text and multimedia data.
- AI-Driven Indexing: Machine learning algorithms can optimize index structures by predicting access patterns and preemptively loading relevant data into memory, reducing query response times.
What is an Approximate Nearest Neighbor (ANN) Search? It’s an algorithm that finds a data point in a data set that’s very close to the given query point, but not necessarily the absolute closest one. An NN algorithm searches exhaustively through all the data to find the perfect match, whereas an ANN algorithm will settle for a match that’s close enough.
3. Automated Data Management and Maintenance
AI-driven databases can automatically adjust configurations and optimize performance based on workload analysis. This includes automatic indexing, query optimization, and resource allocation.
- Adaptive Query Optimization: AI models predict the best execution plans for queries by learning from historical data, continuously improving query performance over time.
Predictive Maintenance: Machine learning models can predict hardware failures and performance degradation, allowing for proactive maintenance and minimizing downtime.
Some examples:
- Azure SQL Database offers built-in AI features such as automatic tuning, which includes automatic indexing and query performance optimization. Azure DBs also provide insights with machine learning to analyze database performance and recommend optimizations.
- Google BigQuery incorporates machine learning to optimize query execution and manage resources efficiently and allows users to create and execute machine learning models directly within the database.
- Amazon Aurora utilizes machine learning to optimize queries, predict database performance issues, and automate database management tasks such as indexing and resource allocation. They also integrate machine learning capabilities directly into the database, allowing for real-time predictions and automated adjustments.
Wrap-Up
The landscape of database technology is rapidly evolving, driven by the need to handle more complex data types, improve performance, and integrate seamlessly with machine learning workflows. Innovations like vectorization during inserts, enhanced indexing techniques, and automated data management are at the forefront of this transformation. As these technologies continue to mature, databases will become even more powerful, enabling new levels of efficiency, intelligence, and security in data management.
Unleash Your Creativity with NVIDIA Jetson Nano: The Ultimate AI Home Lab SBC
If you are looking to practice with machine learning, AI or deep learning without the cloud costs, then look no further than the NVIDIA Jetson Nano, your ticket to unlocking endless possibilities in AI development and innovation. In this quick blog article, we’ll explore some of the remarkable features and benefits of the NVIDIA Jetson Nano and how it can revolutionize your AI projects.
Powerful Performance in a Compact Package
Don’t let its small size fool you – the NVIDIA Jetson Nano packs a punch when it comes to performance. Powered by the NVIDIA CUDA-X AI computing platform, this tiny yet mighty device delivers exceptional processing power for AI workloads. With its quad-core ARM Cortex-A57 CPU and 128-core NVIDIA Maxwell GPU, the Jetson Nano is capable of handling complex AI tasks with ease, whether it’s image recognition, natural language processing, or autonomous navigation.
Here are the full specs: https://developer.nvidia.com/embedded/jetson-nano
One drawback is that the supported image is on Ubuntu 18.04 and there is no support to upgrade.
Easy to Use and Versatile
One of the standout features of the NVIDIA Jetson Nano is its user-friendly design. Whether you’re a seasoned AI developer or a beginner just getting started, the Jetson Nano is incredibly easy to set up and use. Thanks to its comprehensive documentation and extensive developer community, you’ll be up and running in no time, ready to unleash your creativity and bring your AI projects to life.
Here are some links to get started:
https://developer.nvidia.com/embedded/learn/get-started-jetson-nano-devkit
https://developer.nvidia.com/embedded/learn/jetson-ai-certification-programs
Endless Possibilities for Innovation
The NVIDIA Jetson Nano opens up a world of possibilities for AI innovation. Whether you’re building a smart robot, creating intelligent IoT devices, or developing groundbreaking machine learning applications, the Jetson Nano provides the tools and resources you need to succeed. With support for popular AI frameworks like TensorFlow, PyTorch, and Caffe, as well as compatibility with a wide range of sensors and peripherals, the Jetson Nano gives you the freedom to explore and experiment like never before.
Here’s a link to see projects:
https://developer.nvidia.com/embedded/community/jetson-projects?refinementList%5Bworks_with%5D%5B0%5D=Jetson%20Nano%202GB&refinementList%5Bworks_with%5D%5B1%5D=Jetson%20Nano&page=1
Understanding GPU Evolution and Deployment in Datacenter or Cloud
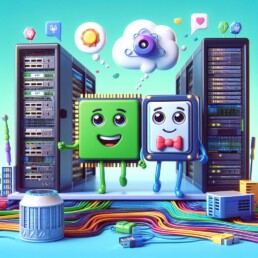
In the current hype of Artificial Intelligence we find ourselves working a lot more with GPUs which is very fun! GPU workloads can be run on physical servers, VMs, or containers. Each approach has its own advantages and disadvantages.
Physical servers offer the highest performance and flexibility, but they are also the most expensive and complex to manage. VMs provide a good balance between performance, flexibility, resource utilization, and cost. Containers offer the best resource utilization and cost savings, but they may sacrifice some performance and isolation.
For Cloud we often recommend Containers since there are services where you can spin up, run your jobs and spin down. A Physical VM running 24-7 on cloud services can be expensive. The performance trade-off is often solved by distributing load or development.
The best deployment option for your GPU workloads will depend on your specific needs and requirements. If you need the highest possible performance and flexibility, physical servers are the way to go. If you are looking to improve resource utilization and cost savings, VMs or containers may be a better option.
Deployment | Description | Benefits | Disadvantages |
---|---|---|---|
Physical servers | Each GPU is directly dedicated to a single workload. | Highest performance and flexibility. | Highest cost and complexity. |
VMs | GPUs can be shared among multiple workloads. | Improved resource utilization and cost savings. | Reduced performance and flexibility. |
Containers | GPUs can be shared among multiple workloads running in the same container host. | Improved resource utilization and cost savings. | Reduced performance and isolation. |
Additional considerations:
- GPU virtualization: GPU virtualization technologies such as NVIDIA vGPU and AMD SR-IOV allow you to share a single physical GPU among multiple VMs or containers. This can improve resource utilization and reduce costs, but it may also reduce performance.
- Workload type: Some GPU workloads are more sensitive to performance than others. For example, machine learning and artificial intelligence workloads often require the highest possible performance. Other workloads, such as video encoding and decoding, may be more tolerant of some performance degradation.
- Licensing: Some GPU software applications are only licensed for use on physical servers. If you need to use this type of software, you will need to deploy your GPU workloads on physical servers.
Overall, the best way to choose the right deployment option for your GPU workloads is to carefully consider your specific needs and requirements.
Here is a table summarizing the key differences and benefits of each deployment options:
Characteristic | Physical server | VM | Container |
---|---|---|---|
Performance | Highest | High | Medium |
Flexibility | Highest | High | Medium |
Resource utilization | Medium | High | Highest |
Cost | Highest | Medium | Lowest |
Complexity | Highest | Medium | Lowest |
Isolation | Highest | High | Medium |
Containers for Data Scientists on top of Azure Container Apps
The Azure Data Science VMs are good for dev and testing and even though you could use a virtual machine scale set, that is a heavy and costly solution.
When thinking about scaling, one good solution is to containerize the Anaconda / Python virtual environments and deploy them to Azure Kubernetes Service or better yet, Azure Container Apps, the new abstraction layer for Kubernetes that Azure provides.
Here is a quick way to create a container with Miniconda 3, Pandas and Jupyter Notebooks to interface with the environment. Here I also show how to deploy this single test container it to Azure Container Apps.
The result:
A Jupyter Notebook with Pandas Running on Azure Container Apps.
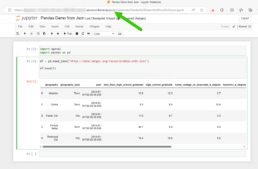
Container Build
If you know the libraries you need then it would make sense to start with the lightest base image which is Miniconda3, you can also deploy the Anaconda3 container but that one might have libraries you might never use that might create unnecessary vulnerabilities top remediate.
Miniconda 3: https://hub.docker.com/r/continuumio/miniconda3
Anaconda 3: https://hub.docker.com/r/continuumio/anaconda3
Below is a simple dockerfile to build a container with pandas, openAi and tensorflow libraries.
FROM continuumio/miniconda3
RUN conda install jupyter -y --quiet && \ mkdir -p /opt/notebooks
WORKDIR /opt/notebooks
RUN pip install pandas
RUN pip install openAI
RUN pip install tensorflow
CMD ["jupyter", "notebook", "--ip='*'", "--port=8888", "--no-browser", "--allow-root"]
Build and Push the Container
Now that you have the container built push it to your registry and deploy it on Azure Container Apps. I use Azure DevOps to get the job done.
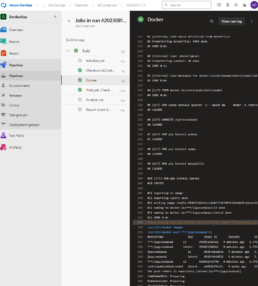
Here’s the pipeline task:
- task: Docker@2
inputs:
containerRegistry: 'dockerRepo'
repository: 'm05tr0/jupycondaoai'
command: 'buildAndPush'
Dockerfile: 'dockerfile'
tags: |
$(Build.BuildId)
latest
Deploy to Azure ContainerApps
Deploying to Azure Container Apps was painless, after understanding the Azure DevOps task, since I can include my ingress configuration in the same step as the container. The only requirement I had to do was configure DNS in my environment. The DevOps task is well documented as well but here’s a link to their official docs.
Architecture / DNS: https://learn.microsoft.com/en-us/azure/container-apps/networking?tabs=azure-cli
Azure Container Apps Deploy Task : https://github.com/microsoft/azure-pipelines-tasks/blob/master/Tasks/AzureContainerAppsV1/README.md
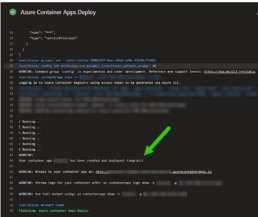
A few things I’d like to point out is that you don’t have to provide a username and password for the container registry the task gets a token from az login. The resource group has to be the one where the Azure Container Apps environment lives, if not a new one will be created. The target port is where the container listens on, see the container build and the jupyter notebooks are pointing to port 8888. If you are using the Container Apps Environment with a private VNET, setting the ingress to external means that the VNET can get to it not outside traffic from the internet. Lastly I disable telemetry to stop reporting.
task: AzureContainerApps@1
inputs:
azureSubscription: 'IngDevOps(XXXXXXXXXXXXXXXXXXXX)'
acrName: 'idocr'
dockerfilePath: 'dockerfile'
imageToBuild: 'idocr.azurecr.io/m05tr0/jupycondaoai'
imageToDeploy: 'idocr.azurecr.io/m05tr0/jupycondaoai'
containerAppName: 'datasci'
resourceGroup: 'IDO-DataScience-Containers'
containerAppEnvironment: 'idoazconapps'
targetPort: '8888'
location: 'East US'
ingress: 'external'
disableTelemetry: true
After deployment I had to get the token which was easy with the Log Stream feature under Monitoring. For a deployment of multiple Jupyter Notebooks it makes sense to use JupyterHub.
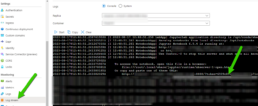