Leveraging GitHub Actions for Efficient Infrastructure Automation with Separate Workflows.
Building infrastructure requires a well-defined pipeline. This article demonstrates how to leverage GitHub Actions to build an Amazon Machine Image (AMI) with Packer and then automatically trigger a separate Terraform workflow via Github’s Workflow API and pass the AMI ID as well.
Benefits:
- Streamlined workflow: Packer builds the AMI, and the AMI ID is seamlessly passed to the Terraform workflow for deployment.
- Reduced manual intervention: The entire process is automated, eliminating the need to manually trigger the Terraform workflow or update the AMI ID.
- Improved efficiency: Faster deployment cycles and reduced risk of errors due to manual configuration.
Why separate workflows?
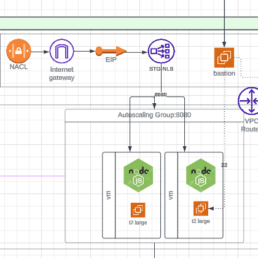
First, think about a simple AWS architecture consisting on a Load Balancer in front of an Autoscaling group, you still need to build a VM image, make sure the load balancer has 2 networks for HA and add security groups for layer 4 access controls. The VM will be built by packer and terraform will deploy the rest of the components so your workflow consists of 2 jobs Packer builds, Terraform deploys but I am here to challenge this approach. You might think this goes against Build / Deploy workflows since most workflows or pipelines have the 2 job pattern of packer build then Terraform deploys but often times we see that we need to separate them because the work we do in Terraform is separate and shouldn’t depend on building an AMI every time.
Think of updating the number of machines on the scale set. Doing it manually will cause drift and the typical workflow will need to run packer before getting to Terraform which is not too bad but we are wasting some cycles.
Separating the workflows makes more sense because you can run terraform to update your infrastructure components from any API Client. Having Terraform in a separate workflow gets rid of the dependency of running packer every time. Ultimately, the choice between the two methods depends on your specific requirements and preferences.
Build and Trigger the Next Workflow
In the packer workflow we add a second job to trigger terraform. We have to pass our Personal Access Token (PAT) and the AMI_ID so that terraform can update the VM Autoscaling Group.
trigger_another_repo:
needs: packer
runs-on: ubuntu-latest
steps:
- name: Trigger second workflow
env:
AMITF: ${{ needs.packer.outputs.AMI_ID_TF }}
run: |
curl -X POST \
-H "Authorization: token ${{ secrets.PAT }}" \
-H "Accept: application/vnd.github.everest-preview+json" \
"https://api.github.com/repos/repo_name/workflow_name/dispatches" \
-d '{"event_type": "trigger_tf_build", "client_payload": {"variable_name": "${{ needs.packer.outputs.AMI_ID_TF }}"}}'
As you can see we are simply using CURL to send the data payload to the Terraform workflow.
The Triggered Workflow Requirements
For the Terraform workflow to start from the packer trigger we need a few simple things.
- Workflow trigger
on:
repository_dispatch:
types: [trigger_prod_tf_build]
- Confirm variable (Optional)
- name: Print Event Payload
run: echo "${{ github.event.client_payload.variable_name }}"
While combining Packer and Terraform into a single workflow can simplify things in certain scenarios, separating them provides more granular control, reusability, and scalability. The best approach depends on the specific needs and complexity of your infrastructure.