Deploying Azure Functions in Containers to Azure Container Apps - like a boss!!!
Introduction
In today's cloud-native world, containerization has become a fundamental approach for deploying applications. Azure Functions can be containerized and deployed to a docker container which means we can deploy them on kubernetes. One compelling option is Azure Container Apps (ACA), which provides a fully managed Kubernetes-based environment with powerful features specifically designed for microservices and containerized applications.
Azure Container Apps is powered by Kubernetes and open-source technologies like Dapr, KEDA, and Envoy. It supports Kubernetes-style apps and microservices with features like service discovery and traffic splitting while enabling event-driven application architectures. This makes it an excellent choice for deploying containerized Azure Functions.
This blog post explores how to deploy Azure Functions in containers to Azure Container Apps, with special focus on the benefits of Envoy for traffic management, revision handling, and logging capabilities for troubleshooting.
Video Demo:
Why Deploy Azure Functions to Container Apps?
Container Apps hosting lets you run your functions in a fully managed, Kubernetes-based environment with built-in support for open-source monitoring, mTLS, Dapr, and Kubernetes Event-driven Autoscaling (KEDA). You can write your function code in any language stack supported by Functions and use the same Functions triggers and bindings with event-driven scaling.
Key advantages include:
- Containerization flexibility: Package your functions with custom dependencies and runtime environments for Dev, QA, STG and PROD
- Kubernetes-based infrastructure: Get the benefits of Kubernetes without managing the complexity
- Microservices architecture support: Deploy functions as part of a larger microservices ecosystem
- Advanced networking: Take advantage of virtual network integration and service discovery
Benefits of Envoy in Azure Container Apps
Azure Container Apps includes a built-in Ingress controller running Envoy. You can use this to expose your application to the outside world and automatically get a URL and an SSL certificate. Envoy brings several significant benefits to your containerized Azure Functions:
1. Advanced Traffic Management
Envoy serves as the backbone of ACA's traffic management capabilities, allowing for:
- Intelligent routing: Route traffic based on paths, headers, and other request attributes
- Load balancing: Distribute traffic efficiently across multiple instances
- Protocol support: Downstream connections support HTTP1.1 and HTTP2, and Envoy automatically detects and upgrades connections if the client connection requires an upgrade.
2. Built-in Security
- TLS termination: Automatic handling of HTTPS traffic with Azure managed certificates
- mTLS support: Azure Container Apps supports peer-to-peer TLS encryption within the environment. Enabling this feature encrypts all network traffic within the environment with a private certificate that is valid within the Azure Container Apps environment scope. Azure Container Apps automatically manage these certificates.
3. Observability
- Detailed metrics and logs for traffic patterns
- Request tracing capabilities
- Performance insights for troubleshooting
Traffic Management for Revisions
One of the most powerful features of Azure Container Apps is its handling of revisions and traffic management between them.
Understanding Revisions
Revisions are immutable snapshots of your container application at a point in time. When you upgrade your container app to a new version, you create a new revision. This allows you to have the old and new versions running simultaneously and use the traffic management functionality to direct traffic to old or new versions of the application.
Traffic Splitting Between Revisions
Traffic split is a mechanism that routes configurable percentages of incoming requests (traffic) to various downstream services. With Azure Container Apps, we can weight traffic between multiple downstream revisions.
This capability enables several powerful deployment strategies:
Blue/Green Deployments
Deploy a new version alongside the existing one, and gradually shift traffic:
- Deploy revision 2 (green) alongside revision 1 (blue)
- Initially direct a small percentage (e.g., 10%) of traffic to revision 2
- Monitor performance and errors
- Gradually increase traffic to revision 2 as confidence grows
- Eventually direct 100% traffic to revision 2
- Retire revision 1 when no longer needed
A/B Testing
Test different implementations with real users:
Traffic splitting is useful for testing updates to your container app. You can use traffic splitting to gradually phase in a new revision in blue-green deployments or in A/B testing. Traffic splitting is based on the weight (percentage) of traffic that is routed to each revision.
Implementation
To implement traffic splitting in Azure Container Apps:
By default, when ingress is enabled, all traffic is routed to the latest deployed revision. When you enable multiple revision mode in your container app, you can split incoming traffic between active revisions.
Here's how to configure it:
- Enable multiple revision mode:
- In the Azure portal, go to your container app
- Select "Revision management"
- Set the mode to "Multiple: Several revisions active simultaneously"
- Apply changes
- Configure traffic weights:
- For each active revision, specify the percentage of traffic it should receive
- Ensure the combined percentage equals 100%
Logging and Troubleshooting
Effective logging is crucial for monitoring and troubleshooting containerized applications. Azure Container Apps provides comprehensive logging capabilities integrated with Azure Monitor.
Centralized Logging Infrastructure
Azure Container Apps environments provide centralized logging capabilities through integration with Azure Monitor and Application Insights. By default, all container apps within an environment send logs to a common Log Analytics workspace, making it easier to query and analyze logs across multiple apps.
Key Logging Benefits
- Unified logging experience: All container apps in an environment send logs to the same workspace
- Detailed container insights: Access container-specific metrics and logs
- Function-specific logging: You can monitor your containerized function app hosted in Container Apps using Azure Monitor Application Insights in the same way you do with apps hosted by Azure Functions.
- Scale event logging: For bindings that support event-driven scaling, scale events are logged as FunctionsScalerInfo and FunctionsScalerError events in your Log Analytics workspace.
Troubleshooting Best Practices
When troubleshooting issues in containerized Azure Functions running on ACA:
- Check application logs: Review function execution logs for errors or exceptions
- Monitor scale events: Identify issues with auto-scaling behavior
- Examine container health: Check for container startup failures or crashes
- Review ingress traffic: Analyze traffic patterns and routing decisions
- Inspect revisions: Verify that traffic is being distributed as expected between revisions
Implementation Steps
Here's the full playlist we did in youtube to follow along: https://www.youtube.com/playlist?list=PLKwr1he0x0Dl2glbE8oHeTgdY-_wZkrhi
In Summary:
- Containerize your Azure Functions app:
- Create a Dockerfile based on the Azure Functions base images
- Build and test your container locally
- Video demo:
- Push your container to a registry:
- Push to Azure Container Registry or another compatible registry
- Create a Container Apps environment:
- Set up the environment with appropriate virtual network and logging settings
- Deploy your function container:
- Use Azure CLI, ARM templates, or the Azure Portal to deploy
- Configure scaling rules, ingress settings, and revision strategy
- Set up traffic management:
- Enable multiple revision mode if desired
- Configure traffic splitting rules for testing or gradual rollout
Conclusion
Deploying Azure Functions in containers to Azure Container Apps combines the best of serverless computing with the flexibility of containers and the rich features of a managed Kubernetes environment. The built-in Envoy proxy provides powerful traffic management capabilities, especially for handling multiple revisions of your application. Meanwhile, the integrated logging infrastructure simplifies monitoring and troubleshooting across all your containerized functions.
This approach is particularly valuable for teams looking to:
- Deploy Azure Functions with custom dependencies
- Integrate functions into a microservices architecture
- Implement sophisticated deployment strategies like blue/green or A/B testing
- Maintain a consistent container-based deployment strategy across all application components
By leveraging these capabilities, you can create more robust, scalable, and manageable serverless applications while maintaining the development simplicity that makes Azure Functions so powerful.
Azure Functions on your local Kubernetes Cluster: A Dev Powerhouse
In today’s fast-paced development landscape, the traditional Dev, QA, STG (Staging), PROD pipeline has become a standard practice. However, the increasing adoption of cloud-based environments has introduced new challenges, particularly in terms of cost and deployment speed. To address these issues, many organizations are exploring strategies to optimize their development and deployment processes. In this article we are exploring the use of our local Kubernetes cluster since Azure Functions can run on containers, this can improve your deployments and cost savings.
KEDA (Kubernetes Event-Driven Autoscaler)
KEDA is a tool that helps manage the scaling of your applications based on the workload they’re handling. Imagine having a website that experiences a sudden surge in traffic. KEDA can automatically increase the number of servers running your website to handle the increased load. Once the traffic subsides, it can also scale down all the way to zero PODS to reduce costs.
What is Scale to Zero? It’s a feature that allows applications to automatically scale down to zero instances when there’s no incoming traffic or activity. This means that the application is essentially turned off to save costs. However, as soon as activity resumes, the application can quickly scale back up to handle the load.
Caveat: Your app needs to be packaged in a way that it can start up fast and not have a high warm-up period.
How Does it Work? KEDA monitors application metrics and automatically scales the number of instances up or down based on predefined rules. KEDA supports a wide range of application metrics that can be used to trigger scaling actions. Here are some examples and the most commonly used ones:
- HTTP Metrics:
- HTTP requests: The number of HTTP requests received by an application.
- HTTP status codes: The frequency of different HTTP status codes returned by an application (e.g., 200, 404, 500).
- Queue Lengths:
- Message queue length: The number of messages waiting to be processed in a message queue.
- Job queue length: The number of jobs waiting to be executed in a job queue.
- Custom Metrics:
- Application-specific metrics: Any custom metrics that can be exposed by your application (e.g., database connection pool size, cache hit rate).
Choosing the right metrics depends on your specific application and scaling needs. For example, if your application relies heavily on message queues, monitoring queue lengths might be the most relevant metric. If your application is CPU-intensive, monitoring CPU utilization could be a good indicator for scaling.
KEDA also supports metric aggregators like Prometheus and StatsD, which can be used to collect and aggregate metrics from various sources and provide a unified view of your application’s performance.
Azure Container Registry
Azure Container Registry (ACR) and Docker Hub are both popular platforms for storing and managing container images. While both offer essential features, Azure Container Registry provides several distinct advantages that make it a compelling choice for many developers and organizations.
Key Benefits of Azure Container Registry
-
Integration with Azure Ecosystem:
- Seamless integration: ACR is deeply integrated with other Azure services, such as Azure Kubernetes Service (AKS), Azure App Service, and Azure Functions. This integration simplifies deployment and management workflows.
- Centralized management: You can manage container images, deployments, and other related resources from a single Azure portal.
-
Enhanced Security and Compliance:
- Private repositories: ACR allows you to create private repositories, ensuring that your container images are not publicly accessible.
- Role-based access control (RBAC): Implement fine-grained access control to manage who can view, create, and modify container images.
- Compliance: ACR meets various industry compliance standards, making it suitable for organizations with strict security requirements.
-
Performance and Scalability:
- Regional proximity: ACR offers multiple regions worldwide, allowing you to store and retrieve images from a location that is geographically closer to your users, improving performance.
- Scalability: ACR can automatically scale to handle increased demand for container images.
-
Advanced Features:
- Webhooks: Trigger custom actions (e.g., build pipelines, notifications) based on events in your registry, such as image pushes or deletes.
- Geo-replication: Replicate your images across multiple regions for improved availability and disaster recovery.
- Integrated vulnerability scanning: Automatically scan your images for known vulnerabilities and receive alerts.
-
Cost-Effective:
- Azure pricing: ACR is part of the Azure ecosystem, allowing you to leverage Azure’s flexible pricing models and potential cost savings through various discounts and promotions.
In summary, while Docker Hub is a valuable platform for sharing container images publicly, Azure Container Registry offers a more comprehensive solution tailored to the needs of organizations that require enhanced security, integration with Azure services, and performance optimization.
ACR and Kubernetes Integration
To pull container images from Azure Container Registry (ACR) in a Kubernetes manifest, you’ll need to add an imagePullSecret
attribute to the relevant deployment or pod specification. This secret stores the credentials required to authenticate with ACR and pull the images.
Here’s a step-by-step guide on how to achieve this:
1. Create a Kubernetes Secret:
- Use the
kubectl create secret docker-registry
command to create a secret that holds your ACR credentials. Replace<your-acr-name>
with the actual name of your ACR instance and<your-acr-password>
with your ACR password:
kubectl create secret docker-registry <your-acr-name> --username=<your-acr-username> --password=<your-acr-password>
2. Reference the Secret in Your Manifest:
- In your Kubernetes manifest (e.g., deployment.yaml, pod.yaml), add the
imagePullSecrets
attribute to thespec
section of the deployment or pod. Reference the name of the secret you created in the previous step:
apiVersion: apps/v1
kind: Deployment
metadata:
name: my-deployment
spec:
replicas: 3
selector:
matchLabels:
app: my-app
template:
metadata:
labels:
app: my-app
spec:
containers:
- name: my-app
image: <your-acr-name>.azurecr.io/<your-image-name>:<your-tag>
imagePullPolicy: Always
imagePullSecrets:
- name: <your-secret-name>
Key Points:
- Replace
<your-acr-name>
,<your-image-name>
,<your-tag>
, and<your-secret-name>
with the appropriate values for your specific ACR instance, image, and secret. - The
imagePullPolicy
is set toAlways
to ensure that the image is always pulled from the registry, even if it’s already present on the node. You can adjust this policy based on your requirements.
Additional Considerations:
- For more complex scenarios, you might consider using service accounts and role-based access control (RBAC) to manage permissions for accessing ACR.
- If you’re using Azure Kubernetes Service (AKS), you can leverage Azure Active Directory (Azure AD) integration for authentication and authorization, simplifying the management of ACR credentials.
By following these steps, you can successfully configure your Kubernetes deployment or pod to pull container images from Azure Container Registry using the imagePullSecret
attribute.
🚀 Mastering Azure Functions in Docker: Secure Your App with Function Keys! 🔒
In this session, we’re merging the robust capabilities of Azure Functions with the versatility of Docker containers.
By the end of this tutorial, you will have a secure and scalable process for deploying your Azure Functions within Docker, equipped with function keys to ensure security.
Why use Azure Functions inside Docker?
Serverless architecture allows you to run code without provisioning or managing servers. Azure Functions take this concept further by providing a fully managed compute platform. Docker, on the other hand, offers a consistent development environment, making it easy to deploy your applications across various environments. Together, they create a robust and efficient way to develop and deploy serverless applications. Later we will be deploy this container to our local kubernetes cluster and to Azure Container Apps.
Development
The Azure Functions Core tools make it easy to package your function into a container with a single command:
func init MyFunctionApp --docker
The command creates the dockerfile and supporting json for your function inside a container and all you need to do is add your code and dependencies. Since we are building a python function we will be adding our python libraries in the requirements.txt
Using Function Keys for Security
Create a host_secret.json
file in the root of your function app directory. Add the following configuration to specify your function key:
{
"masterKey": {
"name": "master",
"value": "your-master-key-here"
},
"functionKeys": {
"default": "your-function-key-here"
}
}
Now this file needs to be added to the container so the function can read it. You can simply add the following to your dockerfile and rebuild:
RUN mkdir /etc/secrets/
ENV FUNCTIONS_SECRETS_PATH=/etc/secrets
ENV AzureWebJobsSecretStorageType=Files
ENV PYTHONHTTPSVERIFY=0
ADD host_secrets.json /etc/secrets/host.json
Testing
Now you can use the function key you set in the previous step as a query parameter for the function’s endpoint in your api client.
Or you can use curl / powershell as well:
curl -X POST \
'http://192.168.1.200:8081/api/getbooks?code=XXXX000something0000XXXX' \
--header 'Accept: */*' \
--header 'User-Agent: Thunder Client (https://www.thunderclient.com)' \
--header 'Content-Type: application/json' \
--data-raw '{
"query": "Dune"
}'
Develop and Test Local Azure Functions from your IDE
Offloading code from apps is a great way to adapt a microservices architecture. If you are still making the decision of whether to create functions or just code on your app, check out the decision matrix article and some gotchas that will help you know if you should create a function or not. Since we have checked the boxes and our code is a great candidate for Azure Functions then here’s our process:
Dev Environment Setup
Azure Functions Core Tools
First thing is to install the Azure Functions core tools on your machine. There are many ways to install the core tools and instructions can be found in the official Microsoft learn doc here: Develop Azure Functions locally using Core Tools | Microsoft Learn . We are using Ubuntu and Python so we did the following:
wget -q https://packages.microsoft.com/config/ubuntu/22.04/packages-microsoft-prod.deb
sudo dpkg -i packages-microsoft-prod.deb
Then:
sudo apt-get update
sudo apt-get install azure-functions-core-tools-4
After getting the core tools you can test by running
func --help
Result:
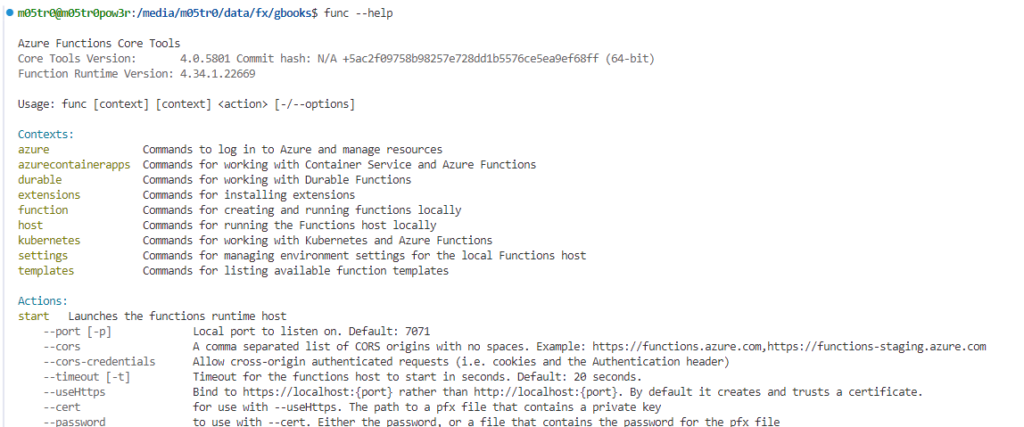
Visual Studio Code Extension
- Go to the Extensions view by clicking the Extensions icon in the Activity Bar.
- Search for “Azure Functions” and install the extension.
- Open the Command Palette (F1) and select
Azure Functions: Install or Update Azure Functions Core Tools
.
Azure Function Fundamentals
Here are some Azure Function Basics. You can write in many languages as described in the official Microsoft learn doc here: Supported Languages with Durable Functions Overview – Azure | Microsoft Learn . We are using Python so here’s our process
I. Create a Python Virtual Environment to manage dependencies:
A Python virtual environment is an isolated environment that allows you to manage dependencies for your project separately from other projects. Here are the key benefits:
- Dependency Isolation:
- Each project can have its own dependencies, regardless of what dependencies other projects have. This prevents conflicts between different versions of packages used in different projects.
- Reproducibility:
- By isolating dependencies, you ensure that your project runs consistently across different environments (development, testing, production). This makes it easier to reproduce bugs and issues.
- Simplified Dependency Management:
- You can easily manage and update dependencies for a specific project without affecting other projects. This is particularly useful when working on multiple projects simultaneously.
- Cleaner Development Environment:
- Your global Python environment remains clean and uncluttered, as all project-specific dependencies are contained within the virtual environment.
Create the virtual environment simply with: python -m venv name_of_venv
What is a Function Route?
A function route is essentially the path part of the URL that maps to your function. When an HTTP request matches this route, the function is executed. Routes are particularly useful for organizing and structuring your API endpoints.
II. Initialization
The line app = func.FunctionApp()
seen in the code snippet below is used in the context of Azure Functions for Python to create an instance of the FunctionApp
class. This instance, app
, serves as the main entry point for defining and managing your Azure Functions within the application. Here’s a breakdown of what it does:
- Initialization:
- It initializes a new
FunctionApp
object, which acts as a container for your function definitions.
- It initializes a new
- Function Registration:
- You use this
app
instance to register your individual functions. Each function is associated with a specific trigger (e.g., HTTP, Timer) and is defined using decorators.
- You use this
import azure.functions as func
app = func.FunctionApp()
@app.function_name(name="HttpTrigger1")
@app.route(route="hello")
def hello_function(req: func.HttpRequest) -> func.HttpResponse:
name = req.params.get('name')
if not name:
try:
req_body = req.get_json()
except ValueError:
pass
else:
name = req_body.get('name')
if name:
return func.HttpResponse(f"Hello, {name}!")
else:
return func.HttpResponse(
"Please pass a name on the query string or in the request body",
status_code=400
)
- The
@app.function_name
and@app.route
decorators are used to define the function’s name and route, respectively. This makes it easy to map HTTP requests to specific functions. - The
hello_function
is defined to handle HTTP requests. It extracts thename
parameter from the query string or request body and returns a greeting. - The function returns an
HttpResponse
object, which is sent back to the client.
What is a Function Route?
A function route is essentially the path part of the URL that maps to your function. When an HTTP request matches this route, the function is executed. Routes are particularly useful for organizing and structuring your API endpoints.
Running The Azure Function
Once you have your code ready to go you can test you function locally by using func start
but there are a few “gotchas” to be aware of:
1. Port Conflicts
- By default,
func start
runs on port 7071. If this port is already in use by another application, you’ll encounter a conflict. You can specify a different port using the--port
option:func start --port 8080
2. Environment Variables
- Ensure that all necessary environment variables are set correctly. Missing or incorrect environment variables can cause your function to fail. You can use a
local.settings.json
file to manage these variables during local development.
3. Dependencies
- Make sure all dependencies listed in your
requirements.txt
(for Python) orpackage.json
(for Node.js) are installed. Missing dependencies can lead to runtime errors.
4. Function Proxies
- If you’re using function proxies, ensure that the
proxies.json
file is correctly configured. Misconfigurations can lead to unexpected behavior or routing issues.
5. Binding Configuration
- Incorrect or incomplete binding configurations in your
function.json
file can cause your function to not trigger as expected. Double-check your bindings to ensure they are set up correctly.
6. Local Settings File
- The
local.settings.json
file should not be checked into source control as it may contain sensitive information. Ensure this file is listed in your.gitignore
file.
7. Cold Start Delays
- When running functions locally, you might experience delays due to cold starts, especially if your function has many dependencies or complex initialization logic.
8. Logging and Monitoring
- Ensure that logging is properly configured to help debug issues. Use the
func start
command’s output to monitor logs and diagnose problems.
9. Version Compatibility
- Ensure that the version of Azure Functions Core Tools you are using is compatible with your function runtime version. Incompatibilities can lead to unexpected errors.
10. Network Issues
- If your function relies on external services or APIs, ensure that your local environment has network access to these services. Network issues can cause your function to fail.
11. File Changes
- Be aware that changes to your function code or configuration files may require restarting the
func start
process to take effect.
12. Debugging
- When debugging, ensure that your IDE is correctly configured to attach to the running function process. Misconfigurations can prevent you from hitting breakpoints.
By keeping these gotchas in mind, you can avoid common pitfalls and ensure a smoother development experience with Azure Functions. If you encounter any specific issues or need further assistance, feel free to ask us!
Testing and Getting Results
If your function starts and you are looking at the logs you will see your endpoints listed as seen below but since you wrote them you know the paths as well and can start testing with your favorite API client, our favorite is Thunder Client.
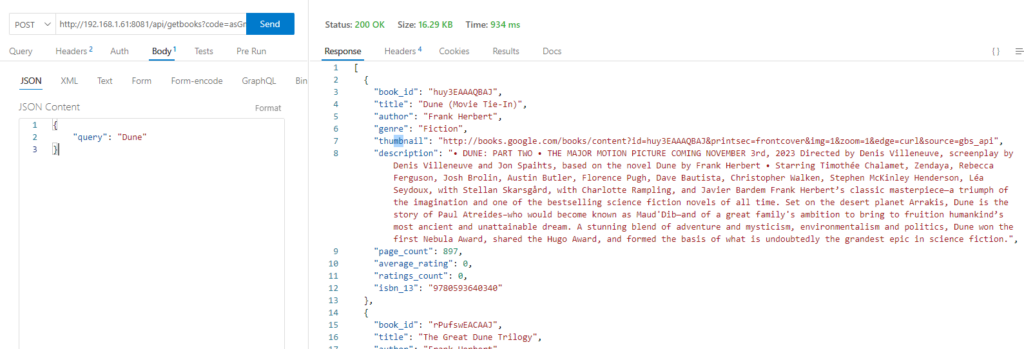
The Response
In Azure Functions, an HTTP response is what your function sends back to the client after processing an HTTP request. Here are the basics:
- Status Code:
- The status code indicates the result of the HTTP request. Common status codes include:
200 OK
: The request was successful.400 Bad Request
: The request was invalid.404 Not Found
: The requested resource was not found.500 Internal Server Error
: An error occurred on the server.
- The status code indicates the result of the HTTP request. Common status codes include:
- Headers:
- HTTP headers provide additional information about the response. Common headers include:
Content-Type
: Specifies the media type of the response (e.g.,application/json
,text/html
).Content-Length
: Indicates the size of the response body.Access-Control-Allow-Origin
: Controls which origins are allowed to access the resource.
- HTTP headers provide additional information about the response. Common headers include:
- Body:
- The body contains the actual data being sent back to the client. This can be in various formats such as JSON, HTML, XML, or plain text. We chose JSON so we can use the different fields and values.
Conclusion
In this article, we’ve explored the process of creating your first Python Azure Function using Visual Studio Code. We covered setting up your environment, including installing Azure Functions Core Tools and the VS Code extension, which simplifies project setup, development, and deployment. We delved into the importance of using a Python virtual environment and a requirements.txt
file for managing dependencies, ensuring consistency, and facilitating collaboration. Additionally, we discussed the basics of function routes and HTTP responses, highlighting how to define routes and customize responses to enhance your API’s structure and usability. By understanding these fundamentals, you can efficiently develop, test, and deploy serverless applications on Azure, leveraging the full potential of Azure Functions. Happy coding!
Django Microservices Approach with Azure Functions on Azure Container Apps
We are creating a multi-part video to explain Azure Functions running on Azure Container Apps so that we can offload some of the code out of our Django App and build our infrastructure with a microservice approach. Here’s part one and below the video a quick high-level explanation for this architecture.
Azure Functions are serverless computing units within Azure that allow you to run event-driven code without having to manage servers. They’re a great choice for building microservices due to their scalability, flexibility, and cost-effectiveness.
Azure Container Apps provide a fully managed platform for deploying and managing containerized applications. By deploying Azure Functions as containerized applications on Container Apps, you gain several advantages:
-
Microservices Architecture:
- Decoupling: Each function becomes an independent microservice, isolated from other parts of your application. This makes it easier to develop, test, and deploy them independently.
- Scalability: You can scale each function individually based on its workload, ensuring optimal resource utilization.
- Resilience: If one microservice fails, the others can continue to operate, improving the overall reliability of your application.
-
Containerization:
- Portability: Containerized functions can be easily moved between environments (development, testing, production) without changes.
- Isolation: Each container runs in its own isolated environment, reducing the risk of conflicts between different functions.
- Efficiency: Containers are optimized for resource utilization, making them ideal for running functions on shared infrastructure.
-
Azure Container Apps Benefits:
- Managed Service: Azure Container Apps handles the underlying infrastructure, allowing you to focus on your application’s logic.
- Scalability: Container Apps automatically scale your functions based on demand, ensuring optimal performance.
- Integration: It seamlessly integrates with other Azure services, such as Azure Functions, Azure App Service, and Azure Kubernetes Service.
In summary, Azure Functions deployed on Azure Container Apps provide a powerful and flexible solution for building microservices. By leveraging the benefits of serverless computing, containerization, and a managed platform, you can create scalable, resilient, and efficient applications.