Deploying Azure Functions in Containers to Azure Container Apps - like a boss!!!
Introduction
In today's cloud-native world, containerization has become a fundamental approach for deploying applications. Azure Functions can be containerized and deployed to a docker container which means we can deploy them on kubernetes. One compelling option is Azure Container Apps (ACA), which provides a fully managed Kubernetes-based environment with powerful features specifically designed for microservices and containerized applications.
Azure Container Apps is powered by Kubernetes and open-source technologies like Dapr, KEDA, and Envoy. It supports Kubernetes-style apps and microservices with features like service discovery and traffic splitting while enabling event-driven application architectures. This makes it an excellent choice for deploying containerized Azure Functions.
This blog post explores how to deploy Azure Functions in containers to Azure Container Apps, with special focus on the benefits of Envoy for traffic management, revision handling, and logging capabilities for troubleshooting.
Video Demo:
Why Deploy Azure Functions to Container Apps?
Container Apps hosting lets you run your functions in a fully managed, Kubernetes-based environment with built-in support for open-source monitoring, mTLS, Dapr, and Kubernetes Event-driven Autoscaling (KEDA). You can write your function code in any language stack supported by Functions and use the same Functions triggers and bindings with event-driven scaling.
Key advantages include:
- Containerization flexibility: Package your functions with custom dependencies and runtime environments for Dev, QA, STG and PROD
- Kubernetes-based infrastructure: Get the benefits of Kubernetes without managing the complexity
- Microservices architecture support: Deploy functions as part of a larger microservices ecosystem
- Advanced networking: Take advantage of virtual network integration and service discovery
Benefits of Envoy in Azure Container Apps
Azure Container Apps includes a built-in Ingress controller running Envoy. You can use this to expose your application to the outside world and automatically get a URL and an SSL certificate. Envoy brings several significant benefits to your containerized Azure Functions:
1. Advanced Traffic Management
Envoy serves as the backbone of ACA's traffic management capabilities, allowing for:
- Intelligent routing: Route traffic based on paths, headers, and other request attributes
- Load balancing: Distribute traffic efficiently across multiple instances
- Protocol support: Downstream connections support HTTP1.1 and HTTP2, and Envoy automatically detects and upgrades connections if the client connection requires an upgrade.
2. Built-in Security
- TLS termination: Automatic handling of HTTPS traffic with Azure managed certificates
- mTLS support: Azure Container Apps supports peer-to-peer TLS encryption within the environment. Enabling this feature encrypts all network traffic within the environment with a private certificate that is valid within the Azure Container Apps environment scope. Azure Container Apps automatically manage these certificates.
3. Observability
- Detailed metrics and logs for traffic patterns
- Request tracing capabilities
- Performance insights for troubleshooting
Traffic Management for Revisions
One of the most powerful features of Azure Container Apps is its handling of revisions and traffic management between them.
Understanding Revisions
Revisions are immutable snapshots of your container application at a point in time. When you upgrade your container app to a new version, you create a new revision. This allows you to have the old and new versions running simultaneously and use the traffic management functionality to direct traffic to old or new versions of the application.
Traffic Splitting Between Revisions
Traffic split is a mechanism that routes configurable percentages of incoming requests (traffic) to various downstream services. With Azure Container Apps, we can weight traffic between multiple downstream revisions.
This capability enables several powerful deployment strategies:
Blue/Green Deployments
Deploy a new version alongside the existing one, and gradually shift traffic:
- Deploy revision 2 (green) alongside revision 1 (blue)
- Initially direct a small percentage (e.g., 10%) of traffic to revision 2
- Monitor performance and errors
- Gradually increase traffic to revision 2 as confidence grows
- Eventually direct 100% traffic to revision 2
- Retire revision 1 when no longer needed
A/B Testing
Test different implementations with real users:
Traffic splitting is useful for testing updates to your container app. You can use traffic splitting to gradually phase in a new revision in blue-green deployments or in A/B testing. Traffic splitting is based on the weight (percentage) of traffic that is routed to each revision.
Implementation
To implement traffic splitting in Azure Container Apps:
By default, when ingress is enabled, all traffic is routed to the latest deployed revision. When you enable multiple revision mode in your container app, you can split incoming traffic between active revisions.
Here's how to configure it:
- Enable multiple revision mode:
- In the Azure portal, go to your container app
- Select "Revision management"
- Set the mode to "Multiple: Several revisions active simultaneously"
- Apply changes
- Configure traffic weights:
- For each active revision, specify the percentage of traffic it should receive
- Ensure the combined percentage equals 100%
Logging and Troubleshooting
Effective logging is crucial for monitoring and troubleshooting containerized applications. Azure Container Apps provides comprehensive logging capabilities integrated with Azure Monitor.
Centralized Logging Infrastructure
Azure Container Apps environments provide centralized logging capabilities through integration with Azure Monitor and Application Insights. By default, all container apps within an environment send logs to a common Log Analytics workspace, making it easier to query and analyze logs across multiple apps.
Key Logging Benefits
- Unified logging experience: All container apps in an environment send logs to the same workspace
- Detailed container insights: Access container-specific metrics and logs
- Function-specific logging: You can monitor your containerized function app hosted in Container Apps using Azure Monitor Application Insights in the same way you do with apps hosted by Azure Functions.
- Scale event logging: For bindings that support event-driven scaling, scale events are logged as FunctionsScalerInfo and FunctionsScalerError events in your Log Analytics workspace.
Troubleshooting Best Practices
When troubleshooting issues in containerized Azure Functions running on ACA:
- Check application logs: Review function execution logs for errors or exceptions
- Monitor scale events: Identify issues with auto-scaling behavior
- Examine container health: Check for container startup failures or crashes
- Review ingress traffic: Analyze traffic patterns and routing decisions
- Inspect revisions: Verify that traffic is being distributed as expected between revisions
Implementation Steps
Here's the full playlist we did in youtube to follow along: https://www.youtube.com/playlist?list=PLKwr1he0x0Dl2glbE8oHeTgdY-_wZkrhi
In Summary:
- Containerize your Azure Functions app:
- Create a Dockerfile based on the Azure Functions base images
- Build and test your container locally
- Video demo:
- Push your container to a registry:
- Push to Azure Container Registry or another compatible registry
- Create a Container Apps environment:
- Set up the environment with appropriate virtual network and logging settings
- Deploy your function container:
- Use Azure CLI, ARM templates, or the Azure Portal to deploy
- Configure scaling rules, ingress settings, and revision strategy
- Set up traffic management:
- Enable multiple revision mode if desired
- Configure traffic splitting rules for testing or gradual rollout
Conclusion
Deploying Azure Functions in containers to Azure Container Apps combines the best of serverless computing with the flexibility of containers and the rich features of a managed Kubernetes environment. The built-in Envoy proxy provides powerful traffic management capabilities, especially for handling multiple revisions of your application. Meanwhile, the integrated logging infrastructure simplifies monitoring and troubleshooting across all your containerized functions.
This approach is particularly valuable for teams looking to:
- Deploy Azure Functions with custom dependencies
- Integrate functions into a microservices architecture
- Implement sophisticated deployment strategies like blue/green or A/B testing
- Maintain a consistent container-based deployment strategy across all application components
By leveraging these capabilities, you can create more robust, scalable, and manageable serverless applications while maintaining the development simplicity that makes Azure Functions so powerful.
Azure Container Apps: Simplifying Container Deployment with Enterprise-Grade Features
In the ever-evolving landscape of cloud computing, organizations are constantly seeking solutions that balance simplicity with enterprise-grade capabilities. Azure Container Apps emerges as a compelling answer to this challenge, offering a powerful abstraction layer over container orchestration while providing the robustness needed for production workloads.
What Makes Azure Container Apps Special?
Azure Container Apps represents Microsoft’s vision for serverless container deployment. While Kubernetes has become the de facto standard for container orchestration, its complexity can be overwhelming for teams that simply want to deploy and scale their containerized applications. Container Apps provides a higher-level abstraction that handles many infrastructure concerns automatically, allowing developers to focus on their applications.
Key Benefits of the Platform
Built-in Load Balancing with Envoy
One of the standout features of Azure Container Apps is its integration with Envoy as a load balancer. This isn’t just any load balancer – Envoy is the same battle-tested proxy used by major cloud-native platforms. It provides:
- Automatic HTTP/2 and gRPC support
- Advanced traffic splitting capabilities for A/B testing
- Built-in circuit breaking and retry logic
- Detailed metrics and tracing
The best part? You don’t need to configure or maintain Envoy yourself. It’s managed entirely by the platform, giving you enterprise-grade load balancing capabilities without the operational overhead.
Integrated Observability with Azure Application Insights
Understanding what’s happening in your containerized applications is crucial for maintaining reliability. Container Apps integrates seamlessly with Azure Application Insights, providing:
- Distributed tracing across your microservices
- Detailed performance metrics and request logging
- Custom metric collection
- Real-time application map visualization
The platform automatically injects the necessary instrumentation, ensuring you have visibility into your applications from day one.
Cost Considerations and Optimization
While Azure Container Apps offers a serverless pricing model that can be cost-effective, it’s important to understand the pricing structure to avoid surprises:
Cost Components
- Compute Usage: Charged per vCPU-second and GB-second of memory used
- Baseline: ~$0.000012/vCPU-second
- Memory: ~$0.000002/GB-second
- Request Processing:
- First 2 million requests/month included
- ~$0.40 per additional million requests
- Storage and Networking:
- Ingress: Free
- Egress: Standard Azure bandwidth rates apply
Cost Optimization Tips
To keep your Azure Container Apps costs under control:
- Right-size your containers by carefully setting resource limits and requests
- Utilize scale-to-zero for non-critical workloads
- Configure appropriate minimum and maximum replica counts
- Monitor and adjust based on actual usage patterns
Advanced Features Worth Exploring
Revision Management
Container Apps introduces a powerful revision management system that allows you to:
- Maintain multiple versions of your application
- Implement blue-green deployments
- Roll back to previous versions if needed
DAPR Integration
For microservices architectures, the built-in DAPR (Distributed Application Runtime) support provides:
- Service-to-service invocation
- State management
- Pub/sub messaging
- Input and output bindings
Conclusion
Azure Container Apps strikes an impressive balance between simplicity and capability. It removes much of the complexity associated with container orchestration while providing the features needed for production-grade applications. Whether you’re building microservices, web applications, or background processing jobs, Container Apps offers a compelling platform that can grow with your needs.
By understanding the pricing model and following best practices for cost optimization, you can leverage this powerful platform while keeping expenses under control. The integration with Azure’s broader ecosystem, particularly Application Insights and Container Registry, creates a seamless experience for developing, deploying, and monitoring containerized applications.
Remember to adjust resource allocations and scaling rules based on your specific workload patterns to optimize both performance and cost. Monitor your application’s metrics through Application Insights to make informed decisions about resource utilization and scaling policies.
Azure Functions on your local Kubernetes Cluster: A Dev Powerhouse
In today’s fast-paced development landscape, the traditional Dev, QA, STG (Staging), PROD pipeline has become a standard practice. However, the increasing adoption of cloud-based environments has introduced new challenges, particularly in terms of cost and deployment speed. To address these issues, many organizations are exploring strategies to optimize their development and deployment processes. In this article we are exploring the use of our local Kubernetes cluster since Azure Functions can run on containers, this can improve your deployments and cost savings.
KEDA (Kubernetes Event-Driven Autoscaler)
KEDA is a tool that helps manage the scaling of your applications based on the workload they’re handling. Imagine having a website that experiences a sudden surge in traffic. KEDA can automatically increase the number of servers running your website to handle the increased load. Once the traffic subsides, it can also scale down all the way to zero PODS to reduce costs.
What is Scale to Zero? It’s a feature that allows applications to automatically scale down to zero instances when there’s no incoming traffic or activity. This means that the application is essentially turned off to save costs. However, as soon as activity resumes, the application can quickly scale back up to handle the load.
Caveat: Your app needs to be packaged in a way that it can start up fast and not have a high warm-up period.
How Does it Work? KEDA monitors application metrics and automatically scales the number of instances up or down based on predefined rules. KEDA supports a wide range of application metrics that can be used to trigger scaling actions. Here are some examples and the most commonly used ones:
- HTTP Metrics:
- HTTP requests: The number of HTTP requests received by an application.
- HTTP status codes: The frequency of different HTTP status codes returned by an application (e.g., 200, 404, 500).
- Queue Lengths:
- Message queue length: The number of messages waiting to be processed in a message queue.
- Job queue length: The number of jobs waiting to be executed in a job queue.
- Custom Metrics:
- Application-specific metrics: Any custom metrics that can be exposed by your application (e.g., database connection pool size, cache hit rate).
Choosing the right metrics depends on your specific application and scaling needs. For example, if your application relies heavily on message queues, monitoring queue lengths might be the most relevant metric. If your application is CPU-intensive, monitoring CPU utilization could be a good indicator for scaling.
KEDA also supports metric aggregators like Prometheus and StatsD, which can be used to collect and aggregate metrics from various sources and provide a unified view of your application’s performance.
Azure Container Registry
Azure Container Registry (ACR) and Docker Hub are both popular platforms for storing and managing container images. While both offer essential features, Azure Container Registry provides several distinct advantages that make it a compelling choice for many developers and organizations.
Key Benefits of Azure Container Registry
-
Integration with Azure Ecosystem:
- Seamless integration: ACR is deeply integrated with other Azure services, such as Azure Kubernetes Service (AKS), Azure App Service, and Azure Functions. This integration simplifies deployment and management workflows.
- Centralized management: You can manage container images, deployments, and other related resources from a single Azure portal.
-
Enhanced Security and Compliance:
- Private repositories: ACR allows you to create private repositories, ensuring that your container images are not publicly accessible.
- Role-based access control (RBAC): Implement fine-grained access control to manage who can view, create, and modify container images.
- Compliance: ACR meets various industry compliance standards, making it suitable for organizations with strict security requirements.
-
Performance and Scalability:
- Regional proximity: ACR offers multiple regions worldwide, allowing you to store and retrieve images from a location that is geographically closer to your users, improving performance.
- Scalability: ACR can automatically scale to handle increased demand for container images.
-
Advanced Features:
- Webhooks: Trigger custom actions (e.g., build pipelines, notifications) based on events in your registry, such as image pushes or deletes.
- Geo-replication: Replicate your images across multiple regions for improved availability and disaster recovery.
- Integrated vulnerability scanning: Automatically scan your images for known vulnerabilities and receive alerts.
-
Cost-Effective:
- Azure pricing: ACR is part of the Azure ecosystem, allowing you to leverage Azure’s flexible pricing models and potential cost savings through various discounts and promotions.
In summary, while Docker Hub is a valuable platform for sharing container images publicly, Azure Container Registry offers a more comprehensive solution tailored to the needs of organizations that require enhanced security, integration with Azure services, and performance optimization.
ACR and Kubernetes Integration
To pull container images from Azure Container Registry (ACR) in a Kubernetes manifest, you’ll need to add an imagePullSecret
attribute to the relevant deployment or pod specification. This secret stores the credentials required to authenticate with ACR and pull the images.
Here’s a step-by-step guide on how to achieve this:
1. Create a Kubernetes Secret:
- Use the
kubectl create secret docker-registry
command to create a secret that holds your ACR credentials. Replace<your-acr-name>
with the actual name of your ACR instance and<your-acr-password>
with your ACR password:
kubectl create secret docker-registry <your-acr-name> --username=<your-acr-username> --password=<your-acr-password>
2. Reference the Secret in Your Manifest:
- In your Kubernetes manifest (e.g., deployment.yaml, pod.yaml), add the
imagePullSecrets
attribute to thespec
section of the deployment or pod. Reference the name of the secret you created in the previous step:
apiVersion: apps/v1
kind: Deployment
metadata:
name: my-deployment
spec:
replicas: 3
selector:
matchLabels:
app: my-app
template:
metadata:
labels:
app: my-app
spec:
containers:
- name: my-app
image: <your-acr-name>.azurecr.io/<your-image-name>:<your-tag>
imagePullPolicy: Always
imagePullSecrets:
- name: <your-secret-name>
Key Points:
- Replace
<your-acr-name>
,<your-image-name>
,<your-tag>
, and<your-secret-name>
with the appropriate values for your specific ACR instance, image, and secret. - The
imagePullPolicy
is set toAlways
to ensure that the image is always pulled from the registry, even if it’s already present on the node. You can adjust this policy based on your requirements.
Additional Considerations:
- For more complex scenarios, you might consider using service accounts and role-based access control (RBAC) to manage permissions for accessing ACR.
- If you’re using Azure Kubernetes Service (AKS), you can leverage Azure Active Directory (Azure AD) integration for authentication and authorization, simplifying the management of ACR credentials.
By following these steps, you can successfully configure your Kubernetes deployment or pod to pull container images from Azure Container Registry using the imagePullSecret
attribute.
🚀 Mastering Azure Functions in Docker: Secure Your App with Function Keys! 🔒
In this session, we’re merging the robust capabilities of Azure Functions with the versatility of Docker containers.
By the end of this tutorial, you will have a secure and scalable process for deploying your Azure Functions within Docker, equipped with function keys to ensure security.
Why use Azure Functions inside Docker?
Serverless architecture allows you to run code without provisioning or managing servers. Azure Functions take this concept further by providing a fully managed compute platform. Docker, on the other hand, offers a consistent development environment, making it easy to deploy your applications across various environments. Together, they create a robust and efficient way to develop and deploy serverless applications. Later we will be deploy this container to our local kubernetes cluster and to Azure Container Apps.
Development
The Azure Functions Core tools make it easy to package your function into a container with a single command:
func init MyFunctionApp --docker
The command creates the dockerfile and supporting json for your function inside a container and all you need to do is add your code and dependencies. Since we are building a python function we will be adding our python libraries in the requirements.txt
Using Function Keys for Security
Create a host_secret.json
file in the root of your function app directory. Add the following configuration to specify your function key:
{
"masterKey": {
"name": "master",
"value": "your-master-key-here"
},
"functionKeys": {
"default": "your-function-key-here"
}
}
Now this file needs to be added to the container so the function can read it. You can simply add the following to your dockerfile and rebuild:
RUN mkdir /etc/secrets/
ENV FUNCTIONS_SECRETS_PATH=/etc/secrets
ENV AzureWebJobsSecretStorageType=Files
ENV PYTHONHTTPSVERIFY=0
ADD host_secrets.json /etc/secrets/host.json
Testing
Now you can use the function key you set in the previous step as a query parameter for the function’s endpoint in your api client.
Or you can use curl / powershell as well:
curl -X POST \
'http://192.168.1.200:8081/api/getbooks?code=XXXX000something0000XXXX' \
--header 'Accept: */*' \
--header 'User-Agent: Thunder Client (https://www.thunderclient.com)' \
--header 'Content-Type: application/json' \
--data-raw '{
"query": "Dune"
}'
Free AI Inference with local Containers that leverage your NVIDIA GPU
First, let’s find out our GPU information from the OS perspective with the following command:
sudo lshw -C display
NVIDIA Drivers
Check your drivers are up to date so you can get the best features and security patches released. We are using ubuntu so will check by first
nvidia-smi
sudo modinfo nvidia | grep version
Then compare to see what’s in the apt repo to see if you have the latest with:
apt-cache search nvidia | grep nvidia-driver-5
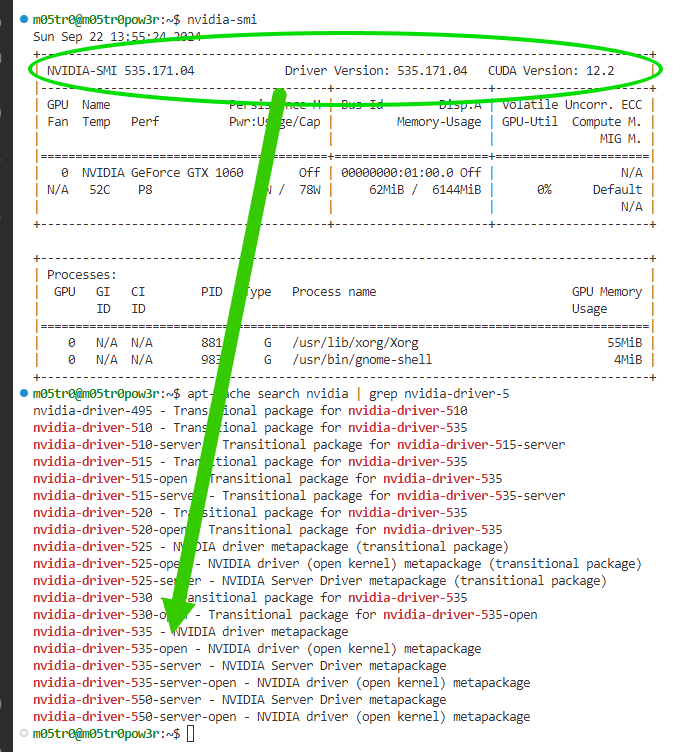
If this is your first time installing drivers please see:
Configure the NVIDIA Toolkit Runtime for Docker
nvidia-ctk is a command-line tool you get when you configure the NVIDIA Container Toolkit. It’s used to configure and manage the container runtime (Docker or containerd) to enable GPU support within containers. To configure you can simply run the following
sudo nvidia-ctk runtime configure --runtime=docker
sudo systemctl restart docker
Here are some of its primary functions:
- Configuring runtime: Modifies the configuration files of Docker or containerd to include the NVIDIA Container Runtime.
- Generating CDI specifications: Creates configuration files for the Container Device Interface (CDI), which allows containers to access GPU devices.
- Listing CDI devices: Lists the available GPU devices that can be used by containers.
In essence, nvidia-ctk acts as a bridge between the container runtime and the NVIDIA GPU, ensuring that containers can effectively leverage GPU acceleration.
Tip: In cases where you want to split one GPU you could create multiple CDI devices which are virtual slices of the GPU. Say you have a GPU with 6GB of RAM, you could create 2 devices with the nvidia-ctk command like so:
nvidia-ctk create-cdi --device-path /dev/nvidia0 --device-id 0 --memory 2G --name cdi1
nvidia-ctk create-cdi --device-path /dev/nvidia0 --device-id 0 --memory 4G --name cdi2
Now you can assign each to containers to limit their utilization of the GPU ram like this:
docker run --gpus device=cdi1,cdi2
Run Containers with GPUs
After configuring the Driver and NVIDIA Container Toolkit you are ready to run GPU-powered containers. One of our favorites is the Ollama containers that allow you to run AI Inference endpoints.
docker run -it --rm --gpus=all -v /home/ollama:/root/.ollama:z -p 11434:11434 --name ollama ollama/ollama
Notice we are using all gpus in this instance.
Sources:
Understanding GPU Evolution and Deployment in Datacenter or Cloud
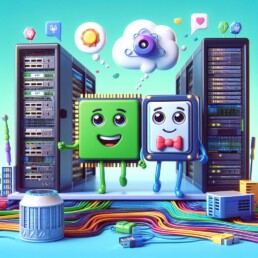
In the current hype of Artificial Intelligence we find ourselves working a lot more with GPUs which is very fun! GPU workloads can be run on physical servers, VMs, or containers. Each approach has its own advantages and disadvantages.
Physical servers offer the highest performance and flexibility, but they are also the most expensive and complex to manage. VMs provide a good balance between performance, flexibility, resource utilization, and cost. Containers offer the best resource utilization and cost savings, but they may sacrifice some performance and isolation.
For Cloud we often recommend Containers since there are services where you can spin up, run your jobs and spin down. A Physical VM running 24-7 on cloud services can be expensive. The performance trade-off is often solved by distributing load or development.
The best deployment option for your GPU workloads will depend on your specific needs and requirements. If you need the highest possible performance and flexibility, physical servers are the way to go. If you are looking to improve resource utilization and cost savings, VMs or containers may be a better option.
Deployment | Description | Benefits | Disadvantages |
---|---|---|---|
Physical servers | Each GPU is directly dedicated to a single workload. | Highest performance and flexibility. | Highest cost and complexity. |
VMs | GPUs can be shared among multiple workloads. | Improved resource utilization and cost savings. | Reduced performance and flexibility. |
Containers | GPUs can be shared among multiple workloads running in the same container host. | Improved resource utilization and cost savings. | Reduced performance and isolation. |
Additional considerations:
- GPU virtualization: GPU virtualization technologies such as NVIDIA vGPU and AMD SR-IOV allow you to share a single physical GPU among multiple VMs or containers. This can improve resource utilization and reduce costs, but it may also reduce performance.
- Workload type: Some GPU workloads are more sensitive to performance than others. For example, machine learning and artificial intelligence workloads often require the highest possible performance. Other workloads, such as video encoding and decoding, may be more tolerant of some performance degradation.
- Licensing: Some GPU software applications are only licensed for use on physical servers. If you need to use this type of software, you will need to deploy your GPU workloads on physical servers.
Overall, the best way to choose the right deployment option for your GPU workloads is to carefully consider your specific needs and requirements.
Here is a table summarizing the key differences and benefits of each deployment options:
Characteristic | Physical server | VM | Container |
---|---|---|---|
Performance | Highest | High | Medium |
Flexibility | Highest | High | Medium |
Resource utilization | Medium | High | Highest |
Cost | Highest | Medium | Lowest |
Complexity | Highest | Medium | Lowest |
Isolation | Highest | High | Medium |
Containers for Data Scientists on top of Azure Container Apps
The Azure Data Science VMs are good for dev and testing and even though you could use a virtual machine scale set, that is a heavy and costly solution.
When thinking about scaling, one good solution is to containerize the Anaconda / Python virtual environments and deploy them to Azure Kubernetes Service or better yet, Azure Container Apps, the new abstraction layer for Kubernetes that Azure provides.
Here is a quick way to create a container with Miniconda 3, Pandas and Jupyter Notebooks to interface with the environment. Here I also show how to deploy this single test container it to Azure Container Apps.
The result:
A Jupyter Notebook with Pandas Running on Azure Container Apps.
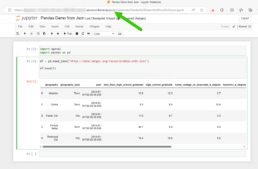
Container Build
If you know the libraries you need then it would make sense to start with the lightest base image which is Miniconda3, you can also deploy the Anaconda3 container but that one might have libraries you might never use that might create unnecessary vulnerabilities top remediate.
Miniconda 3: https://hub.docker.com/r/continuumio/miniconda3
Anaconda 3: https://hub.docker.com/r/continuumio/anaconda3
Below is a simple dockerfile to build a container with pandas, openAi and tensorflow libraries.
FROM continuumio/miniconda3
RUN conda install jupyter -y --quiet && \ mkdir -p /opt/notebooks
WORKDIR /opt/notebooks
RUN pip install pandas
RUN pip install openAI
RUN pip install tensorflow
CMD ["jupyter", "notebook", "--ip='*'", "--port=8888", "--no-browser", "--allow-root"]
Build and Push the Container
Now that you have the container built push it to your registry and deploy it on Azure Container Apps. I use Azure DevOps to get the job done.
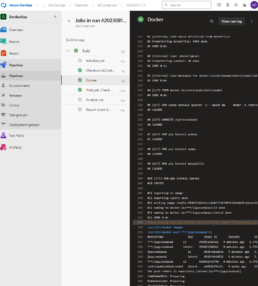
Here’s the pipeline task:
- task: Docker@2
inputs:
containerRegistry: 'dockerRepo'
repository: 'm05tr0/jupycondaoai'
command: 'buildAndPush'
Dockerfile: 'dockerfile'
tags: |
$(Build.BuildId)
latest
Deploy to Azure ContainerApps
Deploying to Azure Container Apps was painless, after understanding the Azure DevOps task, since I can include my ingress configuration in the same step as the container. The only requirement I had to do was configure DNS in my environment. The DevOps task is well documented as well but here’s a link to their official docs.
Architecture / DNS: https://learn.microsoft.com/en-us/azure/container-apps/networking?tabs=azure-cli
Azure Container Apps Deploy Task : https://github.com/microsoft/azure-pipelines-tasks/blob/master/Tasks/AzureContainerAppsV1/README.md
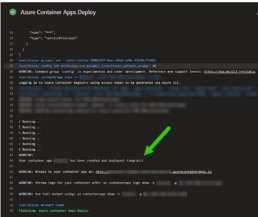
A few things I’d like to point out is that you don’t have to provide a username and password for the container registry the task gets a token from az login. The resource group has to be the one where the Azure Container Apps environment lives, if not a new one will be created. The target port is where the container listens on, see the container build and the jupyter notebooks are pointing to port 8888. If you are using the Container Apps Environment with a private VNET, setting the ingress to external means that the VNET can get to it not outside traffic from the internet. Lastly I disable telemetry to stop reporting.
task: AzureContainerApps@1
inputs:
azureSubscription: 'IngDevOps(XXXXXXXXXXXXXXXXXXXX)'
acrName: 'idocr'
dockerfilePath: 'dockerfile'
imageToBuild: 'idocr.azurecr.io/m05tr0/jupycondaoai'
imageToDeploy: 'idocr.azurecr.io/m05tr0/jupycondaoai'
containerAppName: 'datasci'
resourceGroup: 'IDO-DataScience-Containers'
containerAppEnvironment: 'idoazconapps'
targetPort: '8888'
location: 'East US'
ingress: 'external'
disableTelemetry: true
After deployment I had to get the token which was easy with the Log Stream feature under Monitoring. For a deployment of multiple Jupyter Notebooks it makes sense to use JupyterHub.
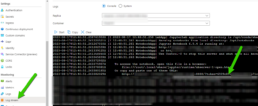
Boosting My Home Lab's Security and Performance with Virtual Apps from Kasm Containers
In the past I’ve worked with VDI solutions like Citrix, VMWare Horizon, Azure Virtual Desktop and others but my favorite is Kasm. For me Kasm has a DevOps friendly and modern way of doing virtual apps and virtual desktops that I didn’t find with other vendors.
With Kasm, apps and desktops run in isolated containers and I can access them easily with my browser, no need to install client software.
Here are my top 3 favorite features:
Boosting My Home Lab's Security and Performance with Virtual Apps from Kasm Containers!
#1 - Runs on the Home Lab!
Kasm Workspaces can be used to create a secure and isolated environment for running applications and browsing the web in your home lab. This can help to protect your devices from malware and other threats.
The community edition is free for 5 concurrent sessions.
If you are a Systems Admin or Engineer you can use it at home for your benefit but also to get familiar with the configuration so that you are better prepared for deploying it at work.
#2 - Low Resource Utilization
Kasm container apps are lightweight and efficient, so they can run quickly and without consuming a lot of resources. This is especially beneficial if you have a limited amount of hardware resources like on a home lab. I run mine in a small ProxMox cluster and offloads work from my main PC. You can also set the amount of compute when configuring your containerized apps.
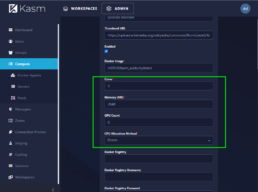
#3 - Security
Each application is run in its own isolated container, which prevents them from interacting with each other or with your PC. This helps to prevent malware or other threats from spreading from one application to another.
The containers could run on isolated Docker networks and with a good firewall solution you can even prevent a self-replicating trojan by segmenting your network and only allowing the necessary ports and traffic flow. Example, if running the Tor Browser containerized app you could only allow it to go outbound to the internet and block SMB (Port 445) from your internal network. If the containerized app gets infected with something like the Emotet Trojan you could be preventing it from spreading further and you could kill the isolated container without having to shutdown or reformatting your local computer.
Code Vulnerability scanning: You can scan your container images in your CI/CD pipelines for vulnerabilities, which helps to identify and fix security weaknesses before you deploy them and before they can be exploited.
Deploy Azure Container Apps with the native AzureRM Terraform provider, no more AzAPI!
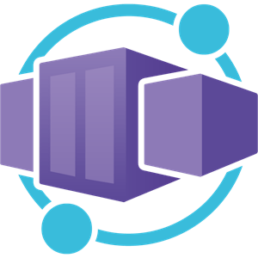
Azure has given us great platforms to run containers. Starting with Azure Container Instance where you can run a small container group just like a docker server and also Azure Kubernetes Service where you can run and manage Kubernetes clusters and containers at scale. Now, the latest Kubernetes abstraction from Azure is called Container Apps!
When a service comes out in a cloud provider their tools are updated right away so when Contrainer Apps came out you could deploy it with ARM or Bicep. You could still deploy it with Terraform by using the AzAPI provider which interacts directly with Azures API but as of a few weeks back (from the publish date of this article) you can use the native AzureRM provider to deploy it.
Code Snippet
resource "azurerm_container_app_environment" "example" {
name = "Example-Environment"
location = azurerm_resource_group.example.location
resource_group_name = azurerm_resource_group.example.name
log_analytics_workspace_id = azurerm_log_analytics_workspace.example.id
}
resource "azurerm_container_app" "example" {
name = "example-app"
container_app_environment_id = azurerm_container_app_environment.example.id
resource_group_name = azurerm_resource_group.example.name
revision_mode = "Single"
template {
container {
name = "examplecontainerapp"
image = "mcr.microsoft.com/azuredocs/containerapps-helloworld:latest"
cpu = 0.25
memory = "0.5Gi"
}
}
}
Sources
Using Github Actions to Build a Kasm Workspace for XChat IRC Client
I really like building and customizing my own Kasm images to use containers to run my applications instead of installing them directly in my computer. Here’s how I built the xchat client Kasm Workspace.
Dockerfile
Most and I mean most of the work is done by the Kasm team since the base images are loaded with all the dependencies needed for Kasm Workspaces and all you have to do is install your app and customize it.
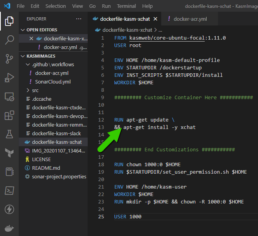
Github Actions for Docker:
- Create a new secret named
DOCKER_HUB_USERNAME
and your Docker ID as value. - Create a new Personal Access Token (PAT) for Docker Hub.
- Add the PAT as a second secret in your GitHub repository, with the name
DOCKER_HUB_ACCESS_TOKEN
.
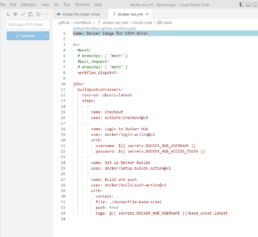
The Github action will run to login, build and push your container to your DockerHub account. Once that’s ready you can proceed to configure kasm to use your container image.
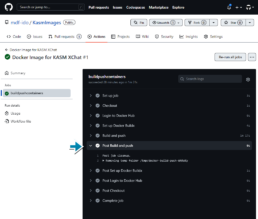
Configuration for Kasm
Once the container is available in the DockerHub repo or other container registries it can be pulled to the Kasm server.
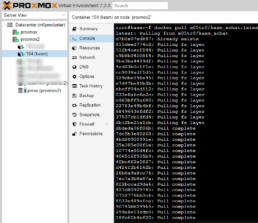
Once the container is in my pulled images I can setup the Kasm Image
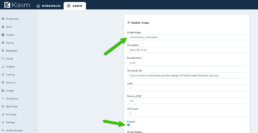
Now it is ready for me to use and further customize.

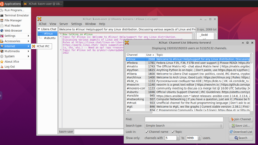