Django Microservices Approach with Azure Functions on Azure Container Apps
We are creating a multi-part video to explain Azure Functions running on Azure Container Apps so that we can offload some of the code out of our Django App and build our infrastructure with a microservice approach. Here’s part one and below the video a quick high-level explanation for this architecture.
Azure Functions are serverless computing units within Azure that allow you to run event-driven code without having to manage servers. They’re a great choice for building microservices due to their scalability, flexibility, and cost-effectiveness.
Azure Container Apps provide a fully managed platform for deploying and managing containerized applications. By deploying Azure Functions as containerized applications on Container Apps, you gain several advantages:
-
Microservices Architecture:
- Decoupling: Each function becomes an independent microservice, isolated from other parts of your application. This makes it easier to develop, test, and deploy them independently.
- Scalability: You can scale each function individually based on its workload, ensuring optimal resource utilization.
- Resilience: If one microservice fails, the others can continue to operate, improving the overall reliability of your application.
-
Containerization:
- Portability: Containerized functions can be easily moved between environments (development, testing, production) without changes.
- Isolation: Each container runs in its own isolated environment, reducing the risk of conflicts between different functions.
- Efficiency: Containers are optimized for resource utilization, making them ideal for running functions on shared infrastructure.
-
Azure Container Apps Benefits:
- Managed Service: Azure Container Apps handles the underlying infrastructure, allowing you to focus on your application’s logic.
- Scalability: Container Apps automatically scale your functions based on demand, ensuring optimal performance.
- Integration: It seamlessly integrates with other Azure services, such as Azure Functions, Azure App Service, and Azure Kubernetes Service.
In summary, Azure Functions deployed on Azure Container Apps provide a powerful and flexible solution for building microservices. By leveraging the benefits of serverless computing, containerization, and a managed platform, you can create scalable, resilient, and efficient applications.
Stay tuned for part 2
Deploying Azure Functions with Azure DevOps: 3 Must-Dos! Code Security Included
Azure Functions is a serverless compute service that allows you to run your code in response to various events, without the need to manage any infrastructure. Azure DevOps, on the other hand, is a set of tools and services that help you build, test, and deploy your applications more efficiently. Combining these two powerful tools can streamline your Azure Functions deployment process and ensure a smooth, automated workflow.
In this blog post, we’ll explore three essential steps to consider when deploying Azure Functions using Azure DevOps.
1. Ensure Consistent Python Versions
When working with Azure Functions, it’s crucial to ensure that the Python version used in your build pipeline matches the Python version configured in your Azure Function. Mismatched versions can lead to unexpected runtime errors and deployment failures.
To ensure consistency, follow these steps:
- Determine the Python version required by your Azure Function. You can find this information in the
requirements.txt
file or thehost.json
file in your Azure Functions project. - In your Azure DevOps pipeline, use the
UsePythonVersion
task to set the Python version to match the one required by your Azure Function.
- task: UsePythonVersion@0
inputs:
versionSpec: '3.9'
addToPath: true
- Verify the Python version in your pipeline by running
python --version
and ensuring it matches the version specified in the previous step.
2. Manage Environment Variables Securely
Azure Functions often require access to various environment variables, such as database connection strings, API keys, or other sensitive information. When deploying your Azure Functions using Azure DevOps, it’s essential to handle these environment variables securely.
Here’s how you can approach this:
- Store your environment variables as Azure DevOps Service Connections or Azure Key Vault Secrets.
- In your Azure DevOps pipeline, use the appropriate task to retrieve and set the environment variables. For example, you can use the
AzureKeyVault
task to fetch secrets from Azure Key Vault.
- task: AzureKeyVault@1
inputs:
azureSubscription: 'Your_Azure_Subscription_Connection'
KeyVaultName: 'your-keyvault-name'
SecretsFilter: '*'
RunAsPreJob: false
- Ensure that your pipeline has the necessary permissions to access the Azure Key Vault or Service Connections.
3. Implement Continuous Integration and Continuous Deployment (CI/CD)
To streamline the deployment process, it’s recommended to set up a CI/CD pipeline in Azure DevOps. This will automatically build, test, and deploy your Azure Functions whenever changes are made to your codebase.
Here’s how you can set up a CI/CD pipeline:
- Create an Azure DevOps Pipeline and configure it to trigger on specific events, such as a push to your repository or a pull request.
- In the pipeline, include steps to build, test, and package your Azure Functions project.
- Add a deployment task to the pipeline to deploy your packaged Azure Functions to the target Azure environment.
# CI/CD pipeline
trigger:
- main
pool:vmImage: ‘ubuntu-latest’
steps:– task: UsePythonVersion@0
inputs:
versionSpec: ‘3.9’
addToPath: true
– script: |pip install -r requirements.txt
displayName: ‘Install dependencies’
– task: AzureWebApp@1inputs:
azureSubscription: ‘Your_Azure_Subscription_Connection’
appName: ‘your-function-app-name’
appType: ‘functionApp’
deployToSlotOrASE: true
resourceGroupName: ‘your-resource-group-name’
slotName: ‘production’
By following these three essential steps, you can ensure a smooth and reliable deployment of your Azure Functions using Azure DevOps, maintaining consistency, security, and automation throughout the process.
Bonus: Embrace DevSecOps with Code Security Checks
As part of your Azure DevOps pipeline, it’s crucial to incorporate security checks to ensure the integrity and safety of your code. This is where the principles of DevSecOps come into play, where security is integrated throughout the software development lifecycle.
Here’s how you can implement code security checks in your Azure DevOps pipeline:
- Use Bandit for Python Code Security: Bandit is a popular open-source tool that analyzes Python code for common security issues. You can integrate Bandit into your Azure DevOps pipeline to automatically scan your Azure Functions code for potential vulnerabilities.
- script: |
pip install bandit
bandit -r your-functions-directory -f custom -o bandit_report.json
displayName: 'Run Bandit Security Scan'
- task: PublishBuildArtifacts@1
inputs:
PathtoPublish: 'bandit_report.json'
ArtifactName: 'bandit-report'
publishLocation: 'Container'
- Leverage the Safety Tool for Dependency Scanning: Safety is another security tool that checks your Python dependencies for known vulnerabilities. Integrate this tool into your Azure DevOps pipeline to ensure that your Azure Functions are using secure dependencies.
- script: |
pip install safety
safety check --full-report
displayName: 'Run Safety Dependency Scan'
- Review Security Scan Results: After running the Bandit and Safety scans, review the generated reports and address any identified security issues before deploying your Azure Functions. You can publish the reports as build artifacts in Azure DevOps for easy access and further investigation.
By incorporating these DevSecOps practices into your Azure DevOps pipeline, you can ensure that your Azure Functions are not only deployed efficiently but also secure and compliant with industry best practices.
Understanding GPU Evolution and Deployment in Datacenter or Cloud
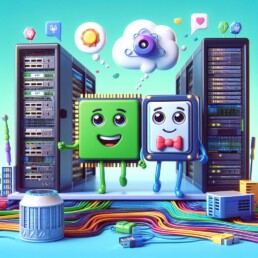
In the current hype of Artificial Intelligence we find ourselves working a lot more with GPUs which is very fun! GPU workloads can be run on physical servers, VMs, or containers. Each approach has its own advantages and disadvantages.
Physical servers offer the highest performance and flexibility, but they are also the most expensive and complex to manage. VMs provide a good balance between performance, flexibility, resource utilization, and cost. Containers offer the best resource utilization and cost savings, but they may sacrifice some performance and isolation.
For Cloud we often recommend Containers since there are services where you can spin up, run your jobs and spin down. A Physical VM running 24-7 on cloud services can be expensive. The performance trade-off is often solved by distributing load or development.
The best deployment option for your GPU workloads will depend on your specific needs and requirements. If you need the highest possible performance and flexibility, physical servers are the way to go. If you are looking to improve resource utilization and cost savings, VMs or containers may be a better option.
Deployment | Description | Benefits | Disadvantages |
---|---|---|---|
Physical servers | Each GPU is directly dedicated to a single workload. | Highest performance and flexibility. | Highest cost and complexity. |
VMs | GPUs can be shared among multiple workloads. | Improved resource utilization and cost savings. | Reduced performance and flexibility. |
Containers | GPUs can be shared among multiple workloads running in the same container host. | Improved resource utilization and cost savings. | Reduced performance and isolation. |
Additional considerations:
- GPU virtualization: GPU virtualization technologies such as NVIDIA vGPU and AMD SR-IOV allow you to share a single physical GPU among multiple VMs or containers. This can improve resource utilization and reduce costs, but it may also reduce performance.
- Workload type: Some GPU workloads are more sensitive to performance than others. For example, machine learning and artificial intelligence workloads often require the highest possible performance. Other workloads, such as video encoding and decoding, may be more tolerant of some performance degradation.
- Licensing: Some GPU software applications are only licensed for use on physical servers. If you need to use this type of software, you will need to deploy your GPU workloads on physical servers.
Overall, the best way to choose the right deployment option for your GPU workloads is to carefully consider your specific needs and requirements.
Here is a table summarizing the key differences and benefits of each deployment options:
Characteristic | Physical server | VM | Container |
---|---|---|---|
Performance | Highest | High | Medium |
Flexibility | Highest | High | Medium |
Resource utilization | Medium | High | Highest |
Cost | Highest | Medium | Lowest |
Complexity | Highest | Medium | Lowest |
Isolation | Highest | High | Medium |
Building Windows Servers with Hashicorp Packer + Terraform on Oracle Cloud Infrastructure OCI
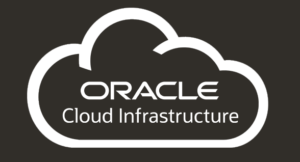
In today’s dynamic IT landscape, platform engineers juggle a diverse array of cloud technologies to cater to specific client needs. Among these, Oracle Cloud Infrastructure (OCI) is rapidly gaining traction due to its competitive pricing for certain services. However, navigating the intricacies of each cloud can present a significant learning curve. This is where cloud-agnostic tools like Terraform and Packer shine. By abstracting away the underlying APIs and automating repetitive tasks, they empower us to leverage OCI’s potential without getting bogged down in vendor-specific complexities.
In this article I show you how to get started with Oracle Cloud by using Packer and Terraform for Windows servers, and this can be used for other Infrastructure as code tasks.
Oracle Cloud Infrastructure Configs
OCI Keys for API Use
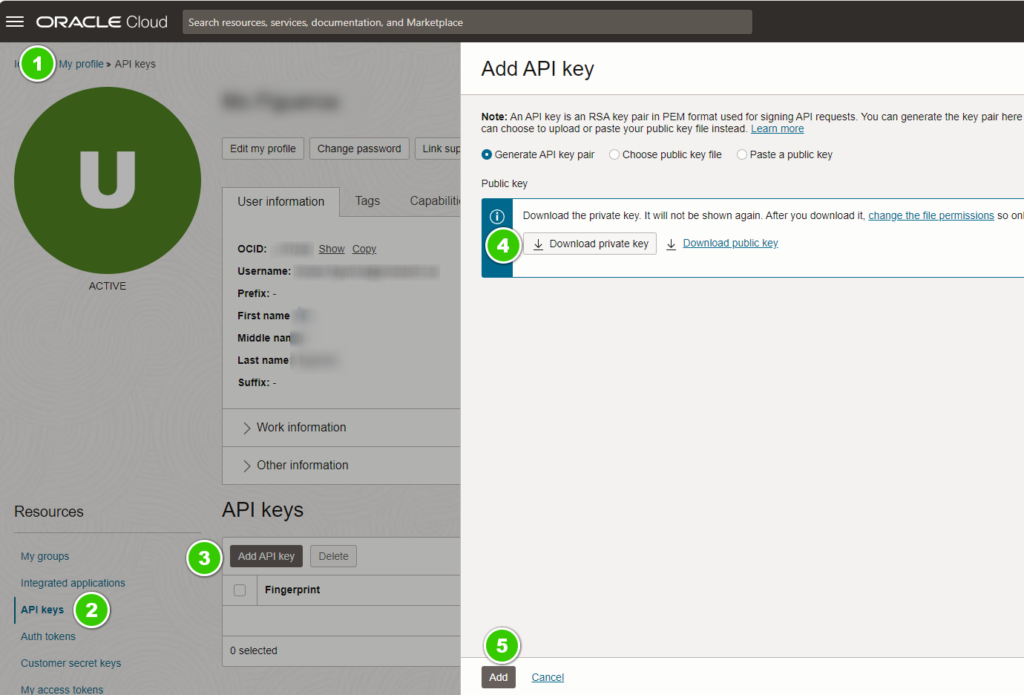
Prerequisite: Before you generate a key pair, create the .oci
directory in your home directory to store the credentials. See SDK and CLI Configuration File for more details.
- View the user’s details:
- If you’re adding an API key for yourself:
Open the Profile menu and click My profile.
- If you’re an administrator adding an API key for another user: Open the navigation menu and click Identity & Security. Under Identity, click Users. Locate the user in the list, and then click the user’s name to view the details.
- If you’re adding an API key for yourself:
- In the Resources section at the bottom left, click API Keys
- Click Add API Key at the top left of the API Keys list. The Add API Key dialog displays.
-
Click Download Private Key and save the key to your
.oci
directory. In most cases, you do not need to download the public key.Note: If your browser downloads the private key to a different directory, be sure to move it to your
.oci
directory. - Click Add.
The key is added and the Configuration File Preview is displayed. The file snippet includes required parameters and values you’ll need to create your configuration file. Copy and paste the configuration file snippet from the text box into your
~/.oci/config file
. (If you have not yet created this file, see SDK and CLI Configuration File for details on how to create one.)After you paste the file contents, you’ll need to update the
key_file
parameter to the location where you saved your private key file.If your config file already has a DEFAULT profile, you’ll need to do one of the following:
- Replace the existing profile and its contents.
- Rename the existing profile.
- Rename this profile to a different name after pasting it into the config file.
- Update the permissions on your downloaded private key file so that only you can view it:
- Go to the
.oci
directory where you placed the private key file. - Use the command
chmod go-rwx ~/.oci/<oci_api_keyfile>.pem
to set the permissions on the fil
- Go to the
Network
Make sure to allow WinRM and RDP so that packer can configure the VM and make it into an image and so that you can RDP to the server after it’s created.
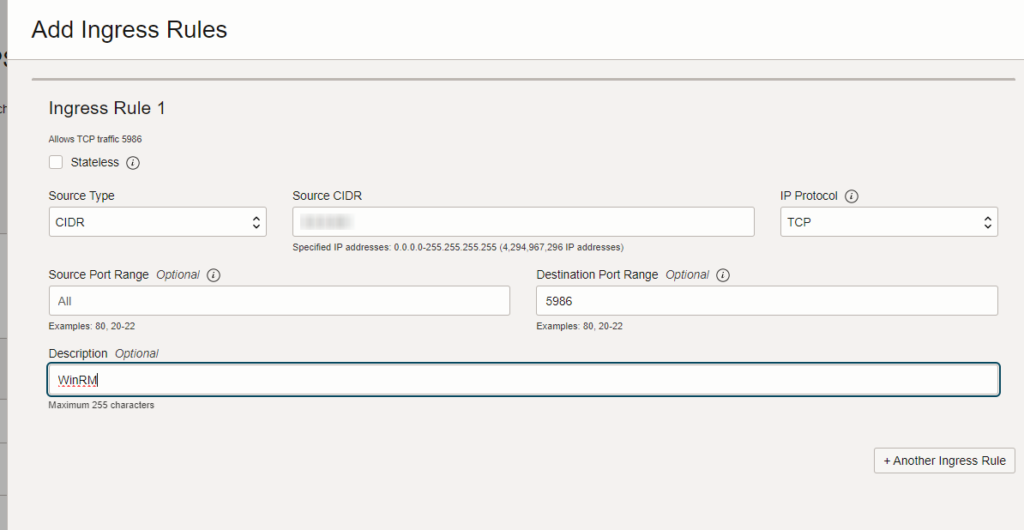
Packer Configuration & Requirements
Install the packer OCI plugin on the host running packer
$ packer plugins install github.com/hashicorp/oracle
Packer Config
- Configure your source
- Availability domain:
oci iam availability-domain list
- Availability domain:
- Get your base image (Drivers Included)
- With the OCI cli:
oci compute image list --compartment-id "ocid#.tenancy.XXXX" --operating-system "Windows" | grep -e 2019 -e ocid1
- With the OCI cli:
- Point to config file that has the OCI Profile we downloaded in the previous steps.
- WinRM Config
- User Data (Bootstrap)
- You must set the password to not be changed at next logon so that packer can connect:
- Code:
#ps1_sysnative
cmd /C 'wmic UserAccount where Name="opc" set PasswordExpires=False'
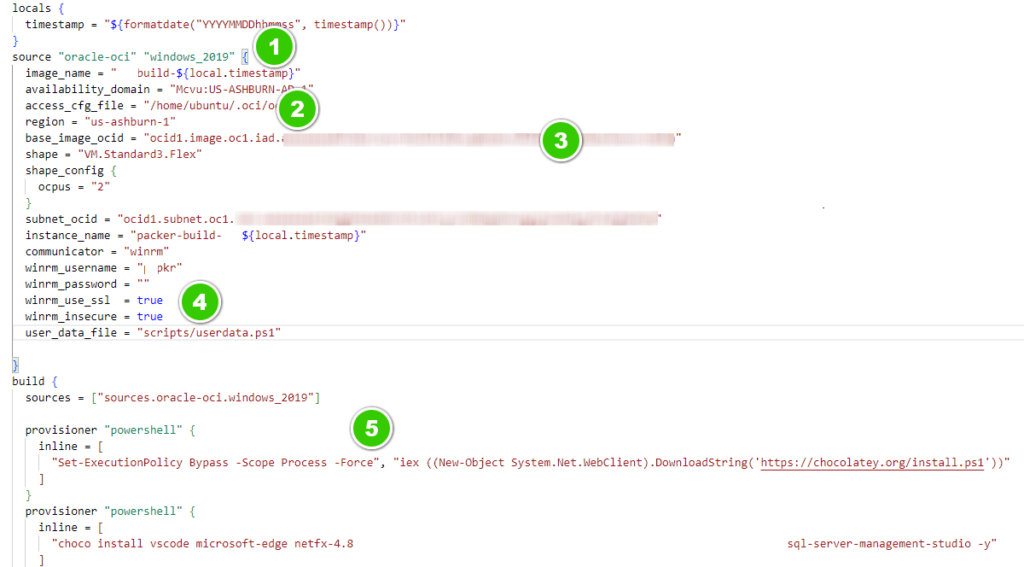
Automating Special Considerations from OCI
Images can be used to launch other instances. The instances launched from these images will include the customizations, configurations, and software installed when the image was created. For windows a we need to sysprep but OCI has specifics on doing so.
Creating a generalized image from an instance will render the instance non-functional, so you should first create a custom image from the instance, and then create a new instance from the custom image. Source below
We automated their instruction by:
- Extract the contents of oracle-cloud_windows-server_generalize_2022-08-24.SED.EXE to your packer scripts directory
- Copy all files to C:\Windows\Panther
- Use the windows-shell provisioner in packer to run Generalize.cmd
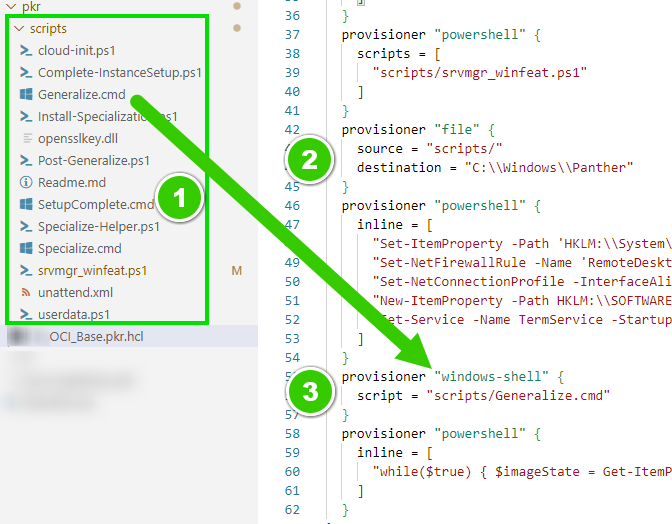
Terraform Config with Oracle Cloud
- Configure the vars
Oracle OCI Terraform Variables - Pass the private key at runtime:
terraform apply --var-file=oci.tfvars -var=private_key_path=~/.oci/user_2024-10-30T10_10_10.478Z.pem
Sources:
Sys-prepping in OCI is specific to their options here’s a link:
https://docs.oracle.com/en-us/iaas/Content/Compute/References/windowsimages.htm#Windows_Generalized_Image_Support_Files
Other Sources:
https://docs.oracle.com/en-us/iaas/Content/API/Concepts/apisigningkey.htm#apisigningkey_topic_How_to_Generate_an_API_Signing_Key_Console
https://github.com/hashicorp/packer/issues/7033
https://github.com/hashicorp/packer-plugin-oracle/tree/main/docs
Avoid Full Downtime on Auto-Scaled Environments by Only Targeting New Instances with Ansible and Github Actions!
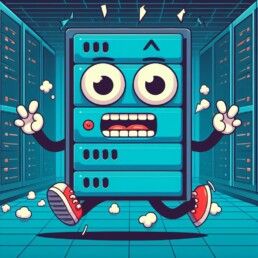
Servers are scared of downtime!
The following Ansible playbook provides a simple but powerful way to compare instance uptime to a threshold “scale_time” variable that you can set in your pipeline variables in Github. By checking the uptime, you can selectively run tasks only on machines newer than that time period you set to avoid downtime on the rest.
Of course the purpose of Ansible is to be idempotent but sometimes during testing we might need to isolate servers to not affect all, specially when using dynamic inventories.
Solution: The Playbook
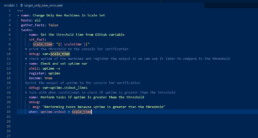
How it works:
- Create a variable in Github Pipeline Variables.
- Set the variable at runtime:
ansible-playbook -i target_only_new_vmss.yml pm2status.yml -e "scaletime=${{ vars.SCALETIME }}"
- The
set_fact
task defines thescale_time
variable based on when the last scaling event occurred. This will be a timestamp. - The
uptime
command gets the current uptime of the instance. This is registered as a variable. - Using a conditional
when
statement, we only run certain tasks if the uptime is less than thescale_time
threshold. - This allows you to selectively target new instances created after the last scale-up event.
Benefits:
- Avoid unnecessary work on stable instances that don’t need updates.
- Focus load and jobs on new machines only
- Safer rollouts in large auto-scaled environments by targeting smaller batches.
- Easy way to check uptime against a set point in time.
Leveraging GitHub Actions for Efficient Infrastructure Automation with Separate Workflows.
Building infrastructure requires a well-defined pipeline. This article demonstrates how to leverage GitHub Actions to build an Amazon Machine Image (AMI) with Packer and then automatically trigger a separate Terraform workflow via Github’s Workflow API and pass the AMI ID as well.
Benefits:
- Streamlined workflow: Packer builds the AMI, and the AMI ID is seamlessly passed to the Terraform workflow for deployment.
- Reduced manual intervention: The entire process is automated, eliminating the need to manually trigger the Terraform workflow or update the AMI ID.
- Improved efficiency: Faster deployment cycles and reduced risk of errors due to manual configuration.
Why separate workflows?
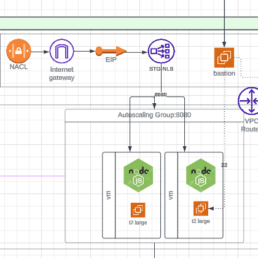
First, think about a simple AWS architecture consisting on a Load Balancer in front of an Autoscaling group, you still need to build a VM image, make sure the load balancer has 2 networks for HA and add security groups for layer 4 access controls. The VM will be built by packer and terraform will deploy the rest of the components so your workflow consists of 2 jobs Packer builds, Terraform deploys but I am here to challenge this approach. You might think this goes against Build / Deploy workflows since most workflows or pipelines have the 2 job pattern of packer build then Terraform deploys but often times we see that we need to separate them because the work we do in Terraform is separate and shouldn’t depend on building an AMI every time.
Think of updating the number of machines on the scale set. Doing it manually will cause drift and the typical workflow will need to run packer before getting to Terraform which is not too bad but we are wasting some cycles.
Separating the workflows makes more sense because you can run terraform to update your infrastructure components from any API Client. Having Terraform in a separate workflow gets rid of the dependency of running packer every time. Ultimately, the choice between the two methods depends on your specific requirements and preferences.
Build and Trigger the Next Workflow
In the packer workflow we add a second job to trigger terraform. We have to pass our Personal Access Token (PAT) and the AMI_ID so that terraform can update the VM Autoscaling Group.
trigger_another_repo:
needs: packer
runs-on: ubuntu-latest
steps:
- name: Trigger second workflow
env:
AMITF: ${{ needs.packer.outputs.AMI_ID_TF }}
run: |
curl -X POST \
-H "Authorization: token ${{ secrets.PAT }}" \
-H "Accept: application/vnd.github.everest-preview+json" \
"https://api.github.com/repos/repo_name/workflow_name/dispatches" \
-d '{"event_type": "trigger_tf_build", "client_payload": {"variable_name": "${{ needs.packer.outputs.AMI_ID_TF }}"}}'
As you can see we are simply using CURL to send the data payload to the Terraform workflow.
The Triggered Workflow Requirements
For the Terraform workflow to start from the packer trigger we need a few simple things.
- Workflow trigger
on:
repository_dispatch:
types: [trigger_prod_tf_build]
- Confirm variable (Optional)
- name: Print Event Payload
run: echo "${{ github.event.client_payload.variable_name }}"
While combining Packer and Terraform into a single workflow can simplify things in certain scenarios, separating them provides more granular control, reusability, and scalability. The best approach depends on the specific needs and complexity of your infrastructure.
Containers for Data Scientists on top of Azure Container Apps
The Azure Data Science VMs are good for dev and testing and even though you could use a virtual machine scale set, that is a heavy and costly solution.
When thinking about scaling, one good solution is to containerize the Anaconda / Python virtual environments and deploy them to Azure Kubernetes Service or better yet, Azure Container Apps, the new abstraction layer for Kubernetes that Azure provides.
Here is a quick way to create a container with Miniconda 3, Pandas and Jupyter Notebooks to interface with the environment. Here I also show how to deploy this single test container it to Azure Container Apps.
The result:
A Jupyter Notebook with Pandas Running on Azure Container Apps.
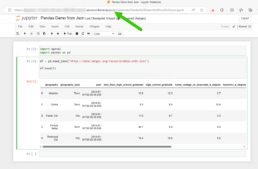
Container Build
If you know the libraries you need then it would make sense to start with the lightest base image which is Miniconda3, you can also deploy the Anaconda3 container but that one might have libraries you might never use that might create unnecessary vulnerabilities top remediate.
Miniconda 3: https://hub.docker.com/r/continuumio/miniconda3
Anaconda 3: https://hub.docker.com/r/continuumio/anaconda3
Below is a simple dockerfile to build a container with pandas, openAi and tensorflow libraries.
FROM continuumio/miniconda3
RUN conda install jupyter -y --quiet && \ mkdir -p /opt/notebooks
WORKDIR /opt/notebooks
RUN pip install pandas
RUN pip install openAI
RUN pip install tensorflow
CMD ["jupyter", "notebook", "--ip='*'", "--port=8888", "--no-browser", "--allow-root"]
Build and Push the Container
Now that you have the container built push it to your registry and deploy it on Azure Container Apps. I use Azure DevOps to get the job done.
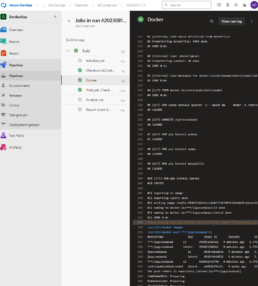
Here’s the pipeline task:
- task: Docker@2
inputs:
containerRegistry: 'dockerRepo'
repository: 'm05tr0/jupycondaoai'
command: 'buildAndPush'
Dockerfile: 'dockerfile'
tags: |
$(Build.BuildId)
latest
Deploy to Azure ContainerApps
Deploying to Azure Container Apps was painless, after understanding the Azure DevOps task, since I can include my ingress configuration in the same step as the container. The only requirement I had to do was configure DNS in my environment. The DevOps task is well documented as well but here’s a link to their official docs.
Architecture / DNS: https://learn.microsoft.com/en-us/azure/container-apps/networking?tabs=azure-cli
Azure Container Apps Deploy Task : https://github.com/microsoft/azure-pipelines-tasks/blob/master/Tasks/AzureContainerAppsV1/README.md
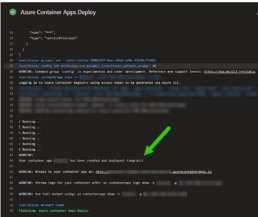
A few things I’d like to point out is that you don’t have to provide a username and password for the container registry the task gets a token from az login. The resource group has to be the one where the Azure Container Apps environment lives, if not a new one will be created. The target port is where the container listens on, see the container build and the jupyter notebooks are pointing to port 8888. If you are using the Container Apps Environment with a private VNET, setting the ingress to external means that the VNET can get to it not outside traffic from the internet. Lastly I disable telemetry to stop reporting.
task: AzureContainerApps@1
inputs:
azureSubscription: 'IngDevOps(XXXXXXXXXXXXXXXXXXXX)'
acrName: 'idocr'
dockerfilePath: 'dockerfile'
imageToBuild: 'idocr.azurecr.io/m05tr0/jupycondaoai'
imageToDeploy: 'idocr.azurecr.io/m05tr0/jupycondaoai'
containerAppName: 'datasci'
resourceGroup: 'IDO-DataScience-Containers'
containerAppEnvironment: 'idoazconapps'
targetPort: '8888'
location: 'East US'
ingress: 'external'
disableTelemetry: true
After deployment I had to get the token which was easy with the Log Stream feature under Monitoring. For a deployment of multiple Jupyter Notebooks it makes sense to use JupyterHub.
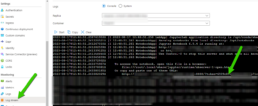
Boosting My Home Lab's Security and Performance with Virtual Apps from Kasm Containers
In the past I’ve worked with VDI solutions like Citrix, VMWare Horizon, Azure Virtual Desktop and others but my favorite is Kasm. For me Kasm has a DevOps friendly and modern way of doing virtual apps and virtual desktops that I didn’t find with other vendors.
With Kasm, apps and desktops run in isolated containers and I can access them easily with my browser, no need to install client software.
Here are my top 3 favorite features:
Boosting My Home Lab's Security and Performance with Virtual Apps from Kasm Containers!
#1 - Runs on the Home Lab!
Kasm Workspaces can be used to create a secure and isolated environment for running applications and browsing the web in your home lab. This can help to protect your devices from malware and other threats.
The community edition is free for 5 concurrent sessions.
If you are a Systems Admin or Engineer you can use it at home for your benefit but also to get familiar with the configuration so that you are better prepared for deploying it at work.
#2 - Low Resource Utilization
Kasm container apps are lightweight and efficient, so they can run quickly and without consuming a lot of resources. This is especially beneficial if you have a limited amount of hardware resources like on a home lab. I run mine in a small ProxMox cluster and offloads work from my main PC. You can also set the amount of compute when configuring your containerized apps.
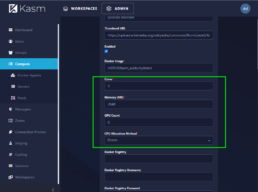
#3 - Security
Each application is run in its own isolated container, which prevents them from interacting with each other or with your PC. This helps to prevent malware or other threats from spreading from one application to another.
The containers could run on isolated Docker networks and with a good firewall solution you can even prevent a self-replicating trojan by segmenting your network and only allowing the necessary ports and traffic flow. Example, if running the Tor Browser containerized app you could only allow it to go outbound to the internet and block SMB (Port 445) from your internal network. If the containerized app gets infected with something like the Emotet Trojan you could be preventing it from spreading further and you could kill the isolated container without having to shutdown or reformatting your local computer.
Code Vulnerability scanning: You can scan your container images in your CI/CD pipelines for vulnerabilities, which helps to identify and fix security weaknesses before you deploy them and before they can be exploited.
Azure Open AI: Private and Secure "ChatGPT like" experience for Enterprises.
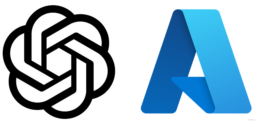
Azure provides the OpenAI service to address the concerns for companies and government agencies that have strong security regulations but want to leverage the power of AI as well.
Most likely you’ve used one of the many AI offerings out there. Open AI’s ChatGPT, Google Bard, Google PaLM with MakerSuite, Perplexity AI, Hugging Chat and many more have been in the latest hype and software companies are racing to integrate them into their products. The main way is to buy a subscription and connect to the ones that offer their API over the internet but as an DevSecOps engineer here’s where the fun starts.
A lot of companies following good security practices block traffic to and from the internet so the first part of all this will be to open the firewall. Next you must protect the credentials of the API user so that it doesn’t get hacked and access will reveal what you are up to. Then you have to trust that OpenAI is not using your data to train their models and that they are keeping your company’s data safe.
It could take a ton of time to plan, design and deploy a secured infrastructure for using large language models and unless you have a very specific use case it might be overkill to build your own.
Here’s a breakdown of a few infrastructure highlights about this service.
3 Main Features
Privacy and Security
Your Chat-GPT like interface called Azure AI Studio runs in your private subscription. It can be linked to one of your VNETs so that you can use internal routing and you can also add private endpoints so that you don’t even have to use it over the internet.
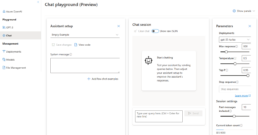
Even if you have to use it over the internet you can lock it down to only allow your public IPs and your developers will need a token for authentication as well that can be scripted to rotate every month.
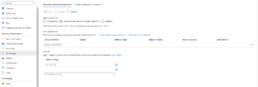
Pricing
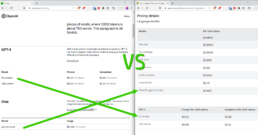
Common Models
- GPT-4 Series: The GPT-4 models are like super-smart computers that can understand and generate human-like text. They can help with things like understanding what people are saying, writing stories or articles, and even translating languages.
Key Differences from GPT-3:
- Model Size: GPT-4 models tend to be larger in terms of parameters compared to GPT-3. Larger models often have more capacity to understand and generate complex text, potentially resulting in improved performance.
- Training Data: GPT-4 models might have been trained on a more extensive and diverse dataset, potentially covering a broader range of topics and languages. This expanded training data can enhance the model’s knowledge and understanding of different subjects.
- Improved Performance: GPT-4 models are likely to demonstrate enhanced performance across various natural language processing tasks. This improvement can include better language comprehension, generating more accurate and coherent text, and understanding context more effectively.
- Fine-tuning Capabilities: GPT-4 might introduce new features or techniques that allow for more efficient fine-tuning of the model. Fine-tuning refers to the process of training a pre-trained model on a specific dataset or task to make it more specialized for that particular use case.
- Contextual Understanding: GPT-4 models might have an improved ability to understand context in a more sophisticated manner. This could allow for a deeper understanding of long passages of text, leading to more accurate responses and better contextual awareness in conversation.
- GPT-3 Base Series: These models are also really smart and can do similar things as GPT-4. They can generate text for writing, help translate languages, complete sentences, and understand how people feel based on what they write.
- Codex Series: The Codex models are designed for programming tasks. They can understand and generate computer code. This helps programmers write code faster, get suggestions for completing code, and even understand and improve existing code.
- Embeddings Series: The Embeddings models are like special tools for understanding text. They can turn words and sentences into numbers that computers can understand. These numbers can be used to do things like classify text into different categories, find information that is similar to what you’re looking for, and even figure out how people feel based on what they write.
Getting Access to it!
Although the service is Generally Available (GA) it is only available in East US and West Europe. You also have to submit an application so that MS can review your company and use case so they can approve or deny your request. This could be due to capacity and for Microsoft to gather information on how companies will be using the service.
The application is here: https://aka.ms/oai/access
Based on research and experience getting this for my clients I always recommend only pick what you initially need and not get too greedy. It would be also wise to speak with your MS Rep and take them out for a beer! For example if you just need code generation then just select the codex option.
Lately getting the service has been easier to get, hopefully soon we won’t need the form and approval dance.

Deploy Azure Container Apps with the native AzureRM Terraform provider, no more AzAPI!
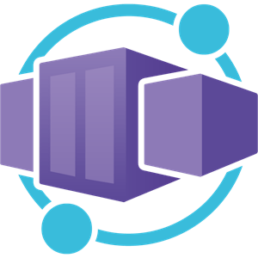
Azure has given us great platforms to run containers. Starting with Azure Container Instance where you can run a small container group just like a docker server and also Azure Kubernetes Service where you can run and manage Kubernetes clusters and containers at scale. Now, the latest Kubernetes abstraction from Azure is called Container Apps!
When a service comes out in a cloud provider their tools are updated right away so when Contrainer Apps came out you could deploy it with ARM or Bicep. You could still deploy it with Terraform by using the AzAPI provider which interacts directly with Azures API but as of a few weeks back (from the publish date of this article) you can use the native AzureRM provider to deploy it.
Code Snippet
resource "azurerm_container_app_environment" "example" {
name = "Example-Environment"
location = azurerm_resource_group.example.location
resource_group_name = azurerm_resource_group.example.name
log_analytics_workspace_id = azurerm_log_analytics_workspace.example.id
}
resource "azurerm_container_app" "example" {
name = "example-app"
container_app_environment_id = azurerm_container_app_environment.example.id
resource_group_name = azurerm_resource_group.example.name
revision_mode = "Single"
template {
container {
name = "examplecontainerapp"
image = "mcr.microsoft.com/azuredocs/containerapps-helloworld:latest"
cpu = 0.25
memory = "0.5Gi"
}
}
}