Deploying Azure Functions in Containers to Azure Container Apps - like a boss!!!
Introduction
In today's cloud-native world, containerization has become a fundamental approach for deploying applications. Azure Functions can be containerized and deployed to a docker container which means we can deploy them on kubernetes. One compelling option is Azure Container Apps (ACA), which provides a fully managed Kubernetes-based environment with powerful features specifically designed for microservices and containerized applications.
Azure Container Apps is powered by Kubernetes and open-source technologies like Dapr, KEDA, and Envoy. It supports Kubernetes-style apps and microservices with features like service discovery and traffic splitting while enabling event-driven application architectures. This makes it an excellent choice for deploying containerized Azure Functions.
This blog post explores how to deploy Azure Functions in containers to Azure Container Apps, with special focus on the benefits of Envoy for traffic management, revision handling, and logging capabilities for troubleshooting.
Video Demo:
Why Deploy Azure Functions to Container Apps?
Container Apps hosting lets you run your functions in a fully managed, Kubernetes-based environment with built-in support for open-source monitoring, mTLS, Dapr, and Kubernetes Event-driven Autoscaling (KEDA). You can write your function code in any language stack supported by Functions and use the same Functions triggers and bindings with event-driven scaling.
Key advantages include:
- Containerization flexibility: Package your functions with custom dependencies and runtime environments for Dev, QA, STG and PROD
- Kubernetes-based infrastructure: Get the benefits of Kubernetes without managing the complexity
- Microservices architecture support: Deploy functions as part of a larger microservices ecosystem
- Advanced networking: Take advantage of virtual network integration and service discovery
Benefits of Envoy in Azure Container Apps
Azure Container Apps includes a built-in Ingress controller running Envoy. You can use this to expose your application to the outside world and automatically get a URL and an SSL certificate. Envoy brings several significant benefits to your containerized Azure Functions:
1. Advanced Traffic Management
Envoy serves as the backbone of ACA's traffic management capabilities, allowing for:
- Intelligent routing: Route traffic based on paths, headers, and other request attributes
- Load balancing: Distribute traffic efficiently across multiple instances
- Protocol support: Downstream connections support HTTP1.1 and HTTP2, and Envoy automatically detects and upgrades connections if the client connection requires an upgrade.
2. Built-in Security
- TLS termination: Automatic handling of HTTPS traffic with Azure managed certificates
- mTLS support: Azure Container Apps supports peer-to-peer TLS encryption within the environment. Enabling this feature encrypts all network traffic within the environment with a private certificate that is valid within the Azure Container Apps environment scope. Azure Container Apps automatically manage these certificates.
3. Observability
- Detailed metrics and logs for traffic patterns
- Request tracing capabilities
- Performance insights for troubleshooting
Traffic Management for Revisions
One of the most powerful features of Azure Container Apps is its handling of revisions and traffic management between them.
Understanding Revisions
Revisions are immutable snapshots of your container application at a point in time. When you upgrade your container app to a new version, you create a new revision. This allows you to have the old and new versions running simultaneously and use the traffic management functionality to direct traffic to old or new versions of the application.
Traffic Splitting Between Revisions
Traffic split is a mechanism that routes configurable percentages of incoming requests (traffic) to various downstream services. With Azure Container Apps, we can weight traffic between multiple downstream revisions.
This capability enables several powerful deployment strategies:
Blue/Green Deployments
Deploy a new version alongside the existing one, and gradually shift traffic:
- Deploy revision 2 (green) alongside revision 1 (blue)
- Initially direct a small percentage (e.g., 10%) of traffic to revision 2
- Monitor performance and errors
- Gradually increase traffic to revision 2 as confidence grows
- Eventually direct 100% traffic to revision 2
- Retire revision 1 when no longer needed
A/B Testing
Test different implementations with real users:
Traffic splitting is useful for testing updates to your container app. You can use traffic splitting to gradually phase in a new revision in blue-green deployments or in A/B testing. Traffic splitting is based on the weight (percentage) of traffic that is routed to each revision.
Implementation
To implement traffic splitting in Azure Container Apps:
By default, when ingress is enabled, all traffic is routed to the latest deployed revision. When you enable multiple revision mode in your container app, you can split incoming traffic between active revisions.
Here's how to configure it:
- Enable multiple revision mode:
- In the Azure portal, go to your container app
- Select "Revision management"
- Set the mode to "Multiple: Several revisions active simultaneously"
- Apply changes
- Configure traffic weights:
- For each active revision, specify the percentage of traffic it should receive
- Ensure the combined percentage equals 100%
Logging and Troubleshooting
Effective logging is crucial for monitoring and troubleshooting containerized applications. Azure Container Apps provides comprehensive logging capabilities integrated with Azure Monitor.
Centralized Logging Infrastructure
Azure Container Apps environments provide centralized logging capabilities through integration with Azure Monitor and Application Insights. By default, all container apps within an environment send logs to a common Log Analytics workspace, making it easier to query and analyze logs across multiple apps.
Key Logging Benefits
- Unified logging experience: All container apps in an environment send logs to the same workspace
- Detailed container insights: Access container-specific metrics and logs
- Function-specific logging: You can monitor your containerized function app hosted in Container Apps using Azure Monitor Application Insights in the same way you do with apps hosted by Azure Functions.
- Scale event logging: For bindings that support event-driven scaling, scale events are logged as FunctionsScalerInfo and FunctionsScalerError events in your Log Analytics workspace.
Troubleshooting Best Practices
When troubleshooting issues in containerized Azure Functions running on ACA:
- Check application logs: Review function execution logs for errors or exceptions
- Monitor scale events: Identify issues with auto-scaling behavior
- Examine container health: Check for container startup failures or crashes
- Review ingress traffic: Analyze traffic patterns and routing decisions
- Inspect revisions: Verify that traffic is being distributed as expected between revisions
Implementation Steps
Here's the full playlist we did in youtube to follow along: https://www.youtube.com/playlist?list=PLKwr1he0x0Dl2glbE8oHeTgdY-_wZkrhi
In Summary:
- Containerize your Azure Functions app:
- Create a Dockerfile based on the Azure Functions base images
- Build and test your container locally
- Video demo:
- Push your container to a registry:
- Push to Azure Container Registry or another compatible registry
- Create a Container Apps environment:
- Set up the environment with appropriate virtual network and logging settings
- Deploy your function container:
- Use Azure CLI, ARM templates, or the Azure Portal to deploy
- Configure scaling rules, ingress settings, and revision strategy
- Set up traffic management:
- Enable multiple revision mode if desired
- Configure traffic splitting rules for testing or gradual rollout
Conclusion
Deploying Azure Functions in containers to Azure Container Apps combines the best of serverless computing with the flexibility of containers and the rich features of a managed Kubernetes environment. The built-in Envoy proxy provides powerful traffic management capabilities, especially for handling multiple revisions of your application. Meanwhile, the integrated logging infrastructure simplifies monitoring and troubleshooting across all your containerized functions.
This approach is particularly valuable for teams looking to:
- Deploy Azure Functions with custom dependencies
- Integrate functions into a microservices architecture
- Implement sophisticated deployment strategies like blue/green or A/B testing
- Maintain a consistent container-based deployment strategy across all application components
By leveraging these capabilities, you can create more robust, scalable, and manageable serverless applications while maintaining the development simplicity that makes Azure Functions so powerful.
OpenWebUI & Ollama: Experience AI on Your Terms with Local Hosting
In an era where AI solutions are behind subscription models behind cloud-based solutions, OpenWebUI and Ollama provide a powerful alternative that prioritize privacy, security, and cost efficiency. These open-source tools are revolutionizing how organizations and individuals can harness AI capabilities while maintaining complete control of models and data used.
Why use local LLMs? #1 Uncensored Models
One significant advantage of local deployment through Ollama is the ability to use a model of your choosing which includes unrestricted LLMs. While cloud-based AI services often implement various limitations and filters on their models to maintain content control and reduce liability, locally hosted models can be used without these restrictions. This provides several benefits:
- Complete control over model behavior and outputs
- Ability to fine-tune models for specific use cases without limitations
- Access to open-source models with different training approaches
- Freedom to experiment with model parameters and configurations
- No artificial constraints on content generation or topic exploration
This flexibility is particularly valuable for research, creative applications, and specialized industry use cases where standard content filters might interfere with legitimate work.
Here’s an amazing article from Eric Hartford on: Uncensored Models
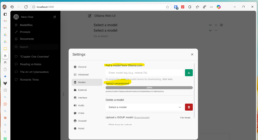
Why use local LLMs? #2 Privacy
When running AI models locally through Ollama and OpenWebUI, all data processing occurs on your own infrastructure. This means:
- Sensitive data never leaves your network perimeter
- No third-party access to your queries or responses
- Complete control over data retention and deletion policies
- Compliance with data sovereignty requirements
- Protection from cloud provider data breaches
Implementation
Requirements:
- Docker
- NVIDIA Container Toolkit (Optional but Recommended)
- GPU + NVIDIA Cuda Installation (Optional but Recommended)
Step 1: Install Ollama
docker run -d -v ollama:/root/.ollama -p 11434:11434 --name ollama ollama/ollama:latest
Step 2: Launch Open WebUI with the new features
docker run -d -p 3000:8080 --add-host=host.docker.internal:host-gateway -v open-webui:/app/backend/data --name open-webui --restart always ghcr.io/open-webui/open-webui:main
Need help setting up Docker and Nvidia Container toolkit?
OpenWeb UI and Ollama
OpenWebUI provides a sophisticated interface for interacting with locally hosted models while maintaining all the security benefits of local deployment. Key features include:
- Intuitive chat interface similar to popular cloud-based AI services
- Support for multiple concurrent model instances
- Built-in prompt templates and history management
- Customizable UI themes and layouts
- API integration capabilities for internal applications
Ollama simplifies the process of running AI models locally while providing robust security features:
- Easy model installation and version management
- Efficient resource utilization through optimized inference
- Support for custom model configurations
- Built-in model verification and integrity checking
- Container-friendly architecture for isolated deployments
Django Microservices Approach with Azure Functions on Azure Container Apps
We are creating a multi-part video to explain Azure Functions running on Azure Container Apps so that we can offload some of the code out of our Django App and build our infrastructure with a microservice approach. Here’s part one and below the video a quick high-level explanation for this architecture.
Azure Functions are serverless computing units within Azure that allow you to run event-driven code without having to manage servers. They’re a great choice for building microservices due to their scalability, flexibility, and cost-effectiveness.
Azure Container Apps provide a fully managed platform for deploying and managing containerized applications. By deploying Azure Functions as containerized applications on Container Apps, you gain several advantages:
-
Microservices Architecture:
- Decoupling: Each function becomes an independent microservice, isolated from other parts of your application. This makes it easier to develop, test, and deploy them independently.
- Scalability: You can scale each function individually based on its workload, ensuring optimal resource utilization.
- Resilience: If one microservice fails, the others can continue to operate, improving the overall reliability of your application.
-
Containerization:
- Portability: Containerized functions can be easily moved between environments (development, testing, production) without changes.
- Isolation: Each container runs in its own isolated environment, reducing the risk of conflicts between different functions.
- Efficiency: Containers are optimized for resource utilization, making them ideal for running functions on shared infrastructure.
-
Azure Container Apps Benefits:
- Managed Service: Azure Container Apps handles the underlying infrastructure, allowing you to focus on your application’s logic.
- Scalability: Container Apps automatically scale your functions based on demand, ensuring optimal performance.
- Integration: It seamlessly integrates with other Azure services, such as Azure Functions, Azure App Service, and Azure Kubernetes Service.
In summary, Azure Functions deployed on Azure Container Apps provide a powerful and flexible solution for building microservices. By leveraging the benefits of serverless computing, containerization, and a managed platform, you can create scalable, resilient, and efficient applications.
Stay tuned for part 2
The AI-Driven Evolution of Databases
The hype of Artificial Intelligence (AI) and Retrieval-Augmented Generation (RAG) is revolutionizing databases and how they are architected. Traditional database management systems (DBMS) are being redefined to harness the capabilities of AI, transforming how data is stored, retrieved, and utilized. In this article I am sharing some of the shifts happening right now to catch up and create better DBs that can play nice with AI.
1. Vectorization and Embedding Integration
Traditional databases store data in structured formats, typically as rows and columns in tables. However, with the rise of AI, there is a need to store and query high-dimensional data such as vectors (embeddings), which represent complex data types like images, audio, and natural language.
- Embedding Vectors: When new data is inserted into the database, it can be vectorized using machine learning models, converting the data into embedding vectors. This allows for efficient similarity searches and comparisons. For example, inserting a new product description could automatically generate an embedding that captures its semantic meaning.
- Vector Databases: Specialized vector databases like Pinecone, Weaviate, FAISS (Facebook AI Similarity Search) and Azure AI Search are designed to handle and index vectorized data, enabling fast and accurate similarity searches and nearest neighbor queries.
A great example is PostgreSQL which can be extended to handle high-dimensional vector data efficiently using the pgvector
extension. This capability is particularly useful for applications involving machine learning, natural language processing, and other AI-driven tasks that rely on vector representations of data.
What is pgvector?
pgvector
is an extension for PostgreSQL that enables the storage, indexing, and querying of vector data. Vectors are often used to represent data in a high-dimensional space, such as word embeddings in NLP, feature vectors in machine learning, and image embeddings in computer vision.
2. Enhanced Indexing Techniques
One of the main changes to support AI is that now your index is required to support ANN (approximate nearest neighbor) queries against vector data. A typical query would be “find me the top N vectors that are most similar to this one”. Each vector may have 100s or 1000s of dimensions, and similarity is based on overall distance across all these dimensions. Your regular btree or hash table index is completely useless for this kind of query, so new types of indexes are provided as part of pgvector on PostgreSQL, or you could use Pinecone, Milvus and many solutions being developed as AI keeps demanding data, these solutions are more specialized for these workloads.
Databases are adopting hybrid indexing techniques that combine traditional indexing methods (B-trees, hash indexes) with AI-driven indexes such as neural hashes and inverted indexes for text and multimedia data.
- AI-Driven Indexing: Machine learning algorithms can optimize index structures by predicting access patterns and preemptively loading relevant data into memory, reducing query response times.
What is an Approximate Nearest Neighbor (ANN) Search? It’s an algorithm that finds a data point in a data set that’s very close to the given query point, but not necessarily the absolute closest one. An NN algorithm searches exhaustively through all the data to find the perfect match, whereas an ANN algorithm will settle for a match that’s close enough.
3. Automated Data Management and Maintenance
AI-driven databases can automatically adjust configurations and optimize performance based on workload analysis. This includes automatic indexing, query optimization, and resource allocation.
- Adaptive Query Optimization: AI models predict the best execution plans for queries by learning from historical data, continuously improving query performance over time.
Predictive Maintenance: Machine learning models can predict hardware failures and performance degradation, allowing for proactive maintenance and minimizing downtime.
Some examples:
- Azure SQL Database offers built-in AI features such as automatic tuning, which includes automatic indexing and query performance optimization. Azure DBs also provide insights with machine learning to analyze database performance and recommend optimizations.
- Google BigQuery incorporates machine learning to optimize query execution and manage resources efficiently and allows users to create and execute machine learning models directly within the database.
- Amazon Aurora utilizes machine learning to optimize queries, predict database performance issues, and automate database management tasks such as indexing and resource allocation. They also integrate machine learning capabilities directly into the database, allowing for real-time predictions and automated adjustments.
Wrap-Up
The landscape of database technology is rapidly evolving, driven by the need to handle more complex data types, improve performance, and integrate seamlessly with machine learning workflows. Innovations like vectorization during inserts, enhanced indexing techniques, and automated data management are at the forefront of this transformation. As these technologies continue to mature, databases will become even more powerful, enabling new levels of efficiency, intelligence, and security in data management.
5 Quick but powerful tips for Dev#$%!Ops Success

There are a ton of variations out there like DevSecOps, MLOps, GitOps (My Favorite), NetOps, DataOps, BizOps, even NoOps, etc. In my opinion, it all comes back to the basic definition which says that Dev<whatever in the middle>OPS is like a soup recipe with 3 main ingredients that are easy to find in your organization's pantry which are people, processes and automation tools. Add the right amount of each ingredient to taste and turn on the heat (Do not cook people)!
The end goal is to serve your customers the best bowl of software soup they've had?!
Create secured, fast and efficient self service software installations for your users by integrating ServiceNow's IntegrationHub, Powershell and Chocolatey

If you have servicenow and chocolatey in your company this is a great project to provide your users with a way to get software without depending on a busy IT admin to remote in to your PC, download files and install apps. Chocolatey steamlines app installs and Service Now takes the same request the user would've submitted to a person and turns it into a powershell script that is securely executed with your mid servers. In this article we show the basics of the integration.