🚀 Mastering Azure Functions in Docker: Secure Your App with Function Keys! 🔒
In this session, we’re merging the robust capabilities of Azure Functions with the versatility of Docker containers.
By the end of this tutorial, you will have a secure and scalable process for deploying your Azure Functions within Docker, equipped with function keys to ensure security.
Why use Azure Functions inside Docker?
Serverless architecture allows you to run code without provisioning or managing servers. Azure Functions take this concept further by providing a fully managed compute platform. Docker, on the other hand, offers a consistent development environment, making it easy to deploy your applications across various environments. Together, they create a robust and efficient way to develop and deploy serverless applications. Later we will be deploy this container to our local kubernetes cluster and to Azure Container Apps.
Development
The Azure Functions Core tools make it easy to package your function into a container with a single command:
func init MyFunctionApp --docker
The command creates the dockerfile and supporting json for your function inside a container and all you need to do is add your code and dependencies. Since we are building a python function we will be adding our python libraries in the requirements.txt
Using Function Keys for Security
Create a host_secret.json
file in the root of your function app directory. Add the following configuration to specify your function key:
{
"masterKey": {
"name": "master",
"value": "your-master-key-here"
},
"functionKeys": {
"default": "your-function-key-here"
}
}
Now this file needs to be added to the container so the function can read it. You can simply add the following to your dockerfile and rebuild:
RUN mkdir /etc/secrets/
ENV FUNCTIONS_SECRETS_PATH=/etc/secrets
ENV AzureWebJobsSecretStorageType=Files
ENV PYTHONHTTPSVERIFY=0
ADD host_secrets.json /etc/secrets/host.json
Testing
Now you can use the function key you set in the previous step as a query parameter for the function’s endpoint in your api client.
Or you can use curl / powershell as well:
curl -X POST \
'http://192.168.1.200:8081/api/getbooks?code=XXXX000something0000XXXX' \
--header 'Accept: */*' \
--header 'User-Agent: Thunder Client (https://www.thunderclient.com)' \
--header 'Content-Type: application/json' \
--data-raw '{
"query": "Dune"
}'
Free AI Inference with local Containers that leverage your NVIDIA GPU
First, let’s find out our GPU information from the OS perspective with the following command:
sudo lshw -C display
NVIDIA Drivers
Check your drivers are up to date so you can get the best features and security patches released. We are using ubuntu so will check by first
nvidia-smi
sudo modinfo nvidia | grep version
Then compare to see what’s in the apt repo to see if you have the latest with:
apt-cache search nvidia | grep nvidia-driver-5
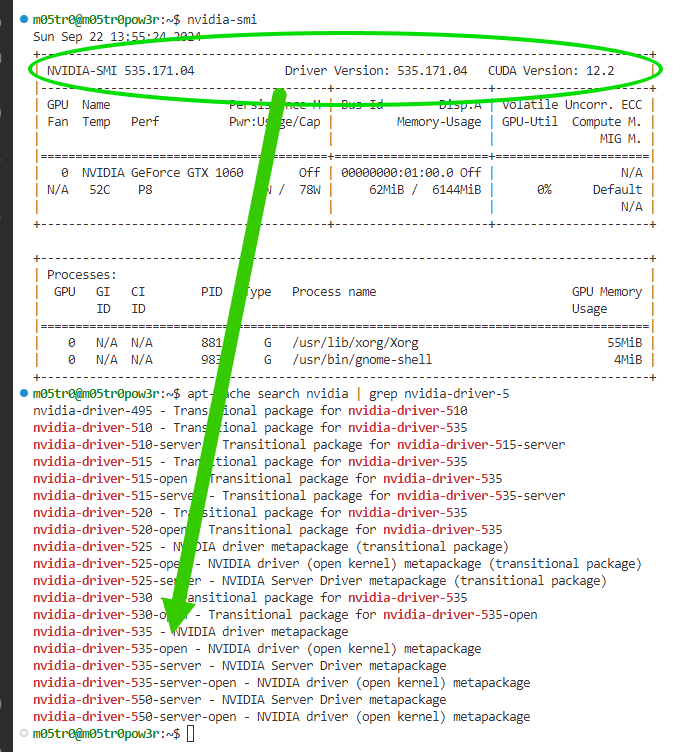
If this is your first time installing drivers please see:
Configure the NVIDIA Toolkit Runtime for Docker
nvidia-ctk is a command-line tool you get when you configure the NVIDIA Container Toolkit. It’s used to configure and manage the container runtime (Docker or containerd) to enable GPU support within containers. To configure you can simply run the following
sudo nvidia-ctk runtime configure --runtime=docker
sudo systemctl restart docker
Here are some of its primary functions:
- Configuring runtime: Modifies the configuration files of Docker or containerd to include the NVIDIA Container Runtime.
- Generating CDI specifications: Creates configuration files for the Container Device Interface (CDI), which allows containers to access GPU devices.
- Listing CDI devices: Lists the available GPU devices that can be used by containers.
In essence, nvidia-ctk acts as a bridge between the container runtime and the NVIDIA GPU, ensuring that containers can effectively leverage GPU acceleration.
Tip: In cases where you want to split one GPU you could create multiple CDI devices which are virtual slices of the GPU. Say you have a GPU with 6GB of RAM, you could create 2 devices with the nvidia-ctk command like so:
nvidia-ctk create-cdi --device-path /dev/nvidia0 --device-id 0 --memory 2G --name cdi1
nvidia-ctk create-cdi --device-path /dev/nvidia0 --device-id 0 --memory 4G --name cdi2
Now you can assign each to containers to limit their utilization of the GPU ram like this:
docker run --gpus device=cdi1,cdi2
Run Containers with GPUs
After configuring the Driver and NVIDIA Container Toolkit you are ready to run GPU-powered containers. One of our favorites is the Ollama containers that allow you to run AI Inference endpoints.
docker run -it --rm --gpus=all -v /home/ollama:/root/.ollama:z -p 11434:11434 --name ollama ollama/ollama
Notice we are using all gpus in this instance.
Sources:
Using Github Actions to Build a Kasm Workspace for XChat IRC Client
I really like building and customizing my own Kasm images to use containers to run my applications instead of installing them directly in my computer. Here’s how I built the xchat client Kasm Workspace.
Dockerfile
Most and I mean most of the work is done by the Kasm team since the base images are loaded with all the dependencies needed for Kasm Workspaces and all you have to do is install your app and customize it.
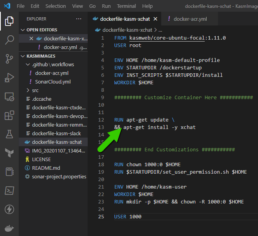
Github Actions for Docker:
- Create a new secret named
DOCKER_HUB_USERNAME
and your Docker ID as value. - Create a new Personal Access Token (PAT) for Docker Hub.
- Add the PAT as a second secret in your GitHub repository, with the name
DOCKER_HUB_ACCESS_TOKEN
.
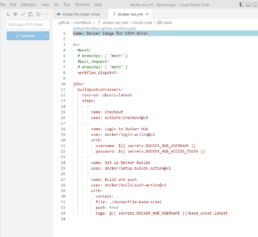
The Github action will run to login, build and push your container to your DockerHub account. Once that’s ready you can proceed to configure kasm to use your container image.
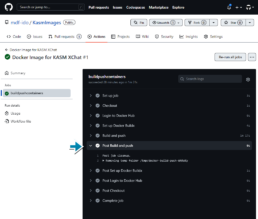
Configuration for Kasm
Once the container is available in the DockerHub repo or other container registries it can be pulled to the Kasm server.
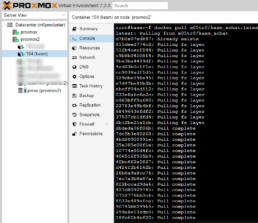
Once the container is in my pulled images I can setup the Kasm Image
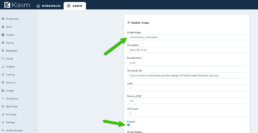
Now it is ready for me to use and further customize.

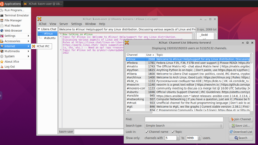